Artificial Intelligence (AI) and Machine Learning (ML) have emerged as transformative technologies with the potential to revolutionize industries across the board. However, despite the hype and promise, the reality of AI implementation tells a sobering story. Recent estimates suggest that more than 80% of AI projects fail – a rate twice as high as that of traditional information technology projects. This alarming statistic underscores the urgent need to understand the challenges in translating AI’s enormous potential into concrete, real-world results.
To shed light on this issue, researchers conducted an in-depth study, interviewing 65 data scientists and engineers with at least five years of experience in building AI/ML models in both industry and academic settings. The findings of this research reveal five primary root causes of AI project failures and offer valuable recommendations for increasing the likelihood of success in both corporate and academic environments.
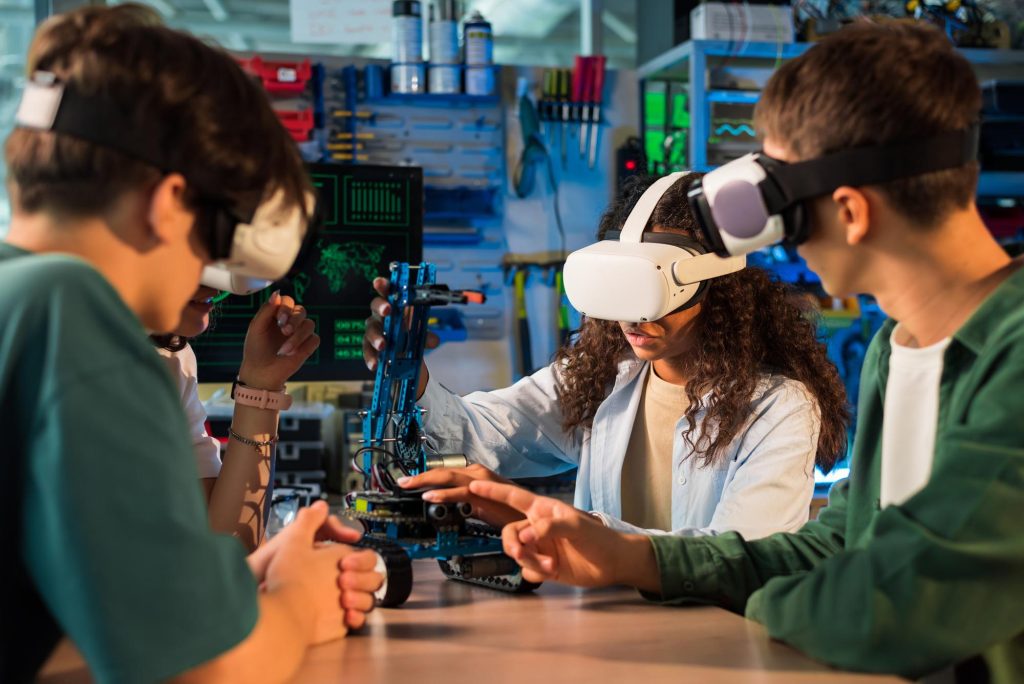
Root Causes of AI Project Failure
- Misunderstanding or Miscommunication of the Problem:
The most prevalent reason for AI project failure stems from a fundamental misalignment between stakeholders regarding the problem that needs to be solved using AI. This miscommunication can occur at various levels within an organization, from C-suite executives to project managers and technical teams. When the project’s purpose and objectives are not clearly defined or understood, it leads to misdirected efforts, wasted resources, and ultimately, project failure. - Inadequate Data:
Many organizations embark on AI projects without possessing the necessary data to adequately train effective AI models. High-quality, relevant, and sufficient data is the lifeblood of any AI system. Without it, even the most sophisticated algorithms will fail to produce meaningful results. This lack of appropriate data can doom a project from the outset, leading to poor performance, biased outcomes, or an inability to solve the intended problem. - Focus on Technology Over Problem-Solving:
In some cases, AI projects fail because organizations become enamored with using the latest and greatest technology rather than focusing on solving real problems for their intended users. This misplaced emphasis on cutting-edge AI techniques can result in impressive demonstrations but impractical solutions that fail to address the core needs of the business or end-users. - Insufficient Infrastructure:
Many organizations lack the necessary infrastructure to effectively manage their data and deploy completed AI models. This deficiency can manifest in various ways, such as inadequate data storage and processing capabilities, lack of robust model versioning systems, or insufficient computing resources for model training and deployment. Without a solid infrastructure foundation, AI projects can face significant hurdles in development, testing, and implementation phases. - Applying AI to Overly Complex Problems:
Sometimes, AI projects fail because the technology is applied to problems that are simply too difficult for current AI capabilities to solve. This overreach often stems from a lack of understanding of AI’s limitations or unrealistic expectations about what AI can achieve. Attempting to tackle problems beyond the scope of current AI technology inevitably leads to project failure and disillusionment with AI’s potential.
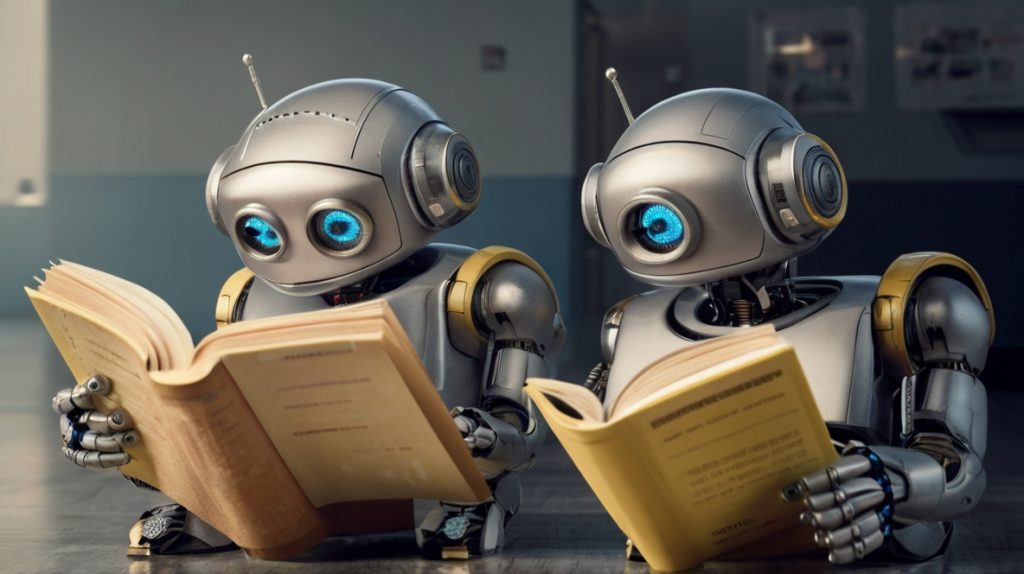
Recommendations for Success
For Industry Leaders:
- Ensure Clear Understanding of Project Purpose and Domain Context:
To address the most common reason for AI project failure, industry leaders must prioritize clear communication about the intent and purpose of each project. This involves ensuring that technical staff have a comprehensive understanding of the business context, user needs, and specific problems the AI solution aims to solve. Regular alignment meetings and cross-functional collaboration can help maintain this shared understanding throughout the project lifecycle. - Choose Enduring Problems:
AI projects require time, patience, and sustained effort to complete successfully. Before initiating any AI project, leaders should be prepared to commit each product team to solving a specific problem for at least a year. This long-term commitment allows for proper data collection, model development, iterative improvements, and thorough testing – all crucial elements for creating robust and effective AI solutions. - Focus on the Problem, Not the Technology:
Successful AI projects maintain a laser focus on the problem to be solved, rather than the specific technology used to solve it. While staying informed about technological advancements is important, the primary emphasis should be on addressing real user needs and business challenges. This problem-centric approach helps ensure that the resulting AI solution delivers tangible value to the organization and its stakeholders. - Invest in Infrastructure:
Up-front investments in infrastructure to support data governance and model deployment can significantly reduce the time required to complete AI projects and increase the volume of high-quality data available for training effective AI models. This includes investing in robust data management systems, scalable computing resources, and tools for model versioning and deployment. A solid infrastructure foundation sets the stage for smoother project execution and more reliable AI systems. - Understand AI’s Limitations:
When considering a potential AI project, leaders need to include technical experts in assessing the project’s feasibility. This involves a realistic evaluation of current AI capabilities, the complexity of the problem at hand, and the resources required for successful implementation. By understanding AI’s limitations, organizations can avoid embarking on projects that are doomed to fail due to technological constraints.
For Academia Leaders:
- Overcome Data-Collection Barriers through Partnerships with Government:
To address the challenge of limited access to high-quality data, academic institutions should forge partnerships with government agencies. These collaborations can provide researchers with access to data of the provenance needed for rigorous academic research, enabling more meaningful and impactful AI studies. - Expand Doctoral Programs in Data Science for Practitioners:
Computer science and data science program leaders should learn from disciplines such as international relations, where practitioner doctoral programs often exist alongside traditional research-focused programs. By creating similar pathways in AI and data science, universities can bridge the gap between academic research and practical application, enabling researchers to apply their findings to urgent real-world problems.
The Broader Context of AI Project Challenges
Beyond these specific root causes and recommendations, it’s crucial to understand the unique challenges that AI projects face compared to traditional software development:
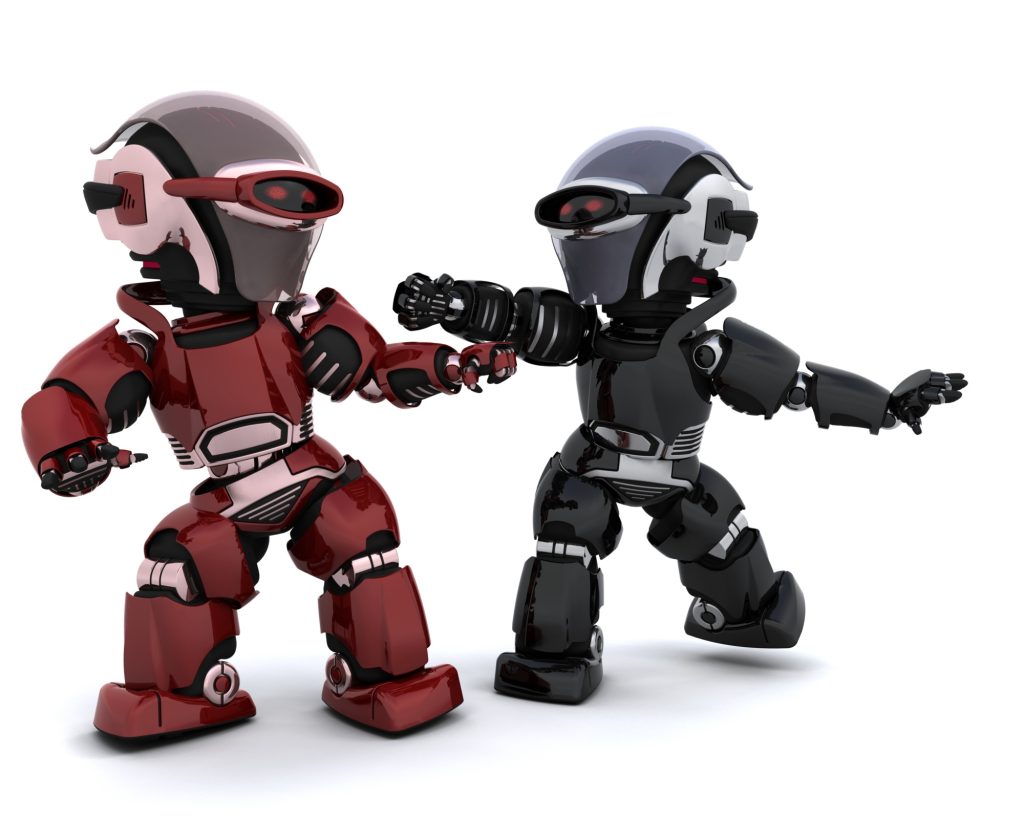
- Indeterministic Outcomes:
Unlike conventional software systems that follow predetermined logic, AI systems often produce results that are not entirely predictable. This inherent uncertainty can make it difficult to guarantee specific performance metrics or outcomes, complicating project planning and stakeholder expectations. - Capability Uncertainty:
The full capabilities and limitations of AI systems may not become apparent until they are deployed and used in real-world scenarios. This uncertainty can lead to unexpected challenges during implementation and may require significant adjustments post-deployment. - Unintended Consequences:
AI deployments can sometimes lead to unexpected and potentially harmful outcomes. These unintended consequences can damage user trust, harm an organization’s reputation, and even have broader societal impacts. Addressing these issues often requires ongoing monitoring, adjustment, and ethical considerations throughout the AI system’s lifecycle. - Cost and ROI Justification:
The upfront costs of AI projects can be substantial, encompassing data collection and preparation, infrastructure investments, and specialized talent acquisition. Moreover, the return on investment (ROI) for AI projects may be challenging to quantify, especially in the short term. This difficulty in demonstrating immediate financial benefits can make it hard to secure long-term support and funding for AI initiatives. - Scalability and Maintenance Challenges:
As AI systems grow and evolve, maintaining and scaling them can become increasingly complex. This includes managing larger datasets, adapting to changing environments, and ensuring consistent performance across different use cases and user groups.
These challenges apply across a wide range of AI applications, including but not limited to recommender systems, self-driving vehicles, computer vision technologies, healthcare diagnostics, autonomous robots, and financial risk assessment tools. Each application domain brings its own set of specific challenges, further complicating the landscape of AI project implementation.
As artificial intelligence continues to evolve and mature, addressing these root causes of failure and implementing the recommended strategies will be crucial for organizations seeking to harness the power of AI. By focusing on clear communication, problem-solving, infrastructure development, and realistic expectations, both industry and academia can improve the success rate of AI projects and unlock the transformative potential of this technology.
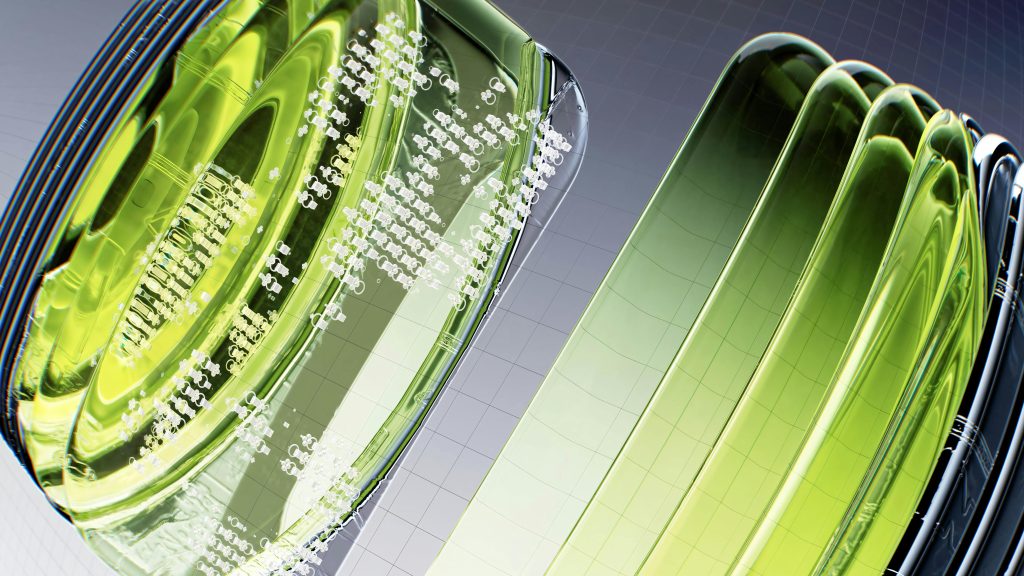
The path to successful AI implementation is not without obstacles, but with a thoughtful approach, organizations can navigate these challenges. By learning from past failures, fostering collaboration between industry and academia, and maintaining a steadfast focus on solving real-world problems, we can work towards realizing the full potential of artificial intelligence in driving innovation and progress across various sectors of society.
Copyright©dhaka.ai
tags: Artificial Intelligence, Ai, Dhaka Ai, Ai In Bangladesh, Ai In Dhaka, Future of AI, Artificial Intelligence in Bangladesh