Quantum computing is an exciting new paradigm of computation that harnesses the strange properties of quantum mechanics to perform calculations exponentially faster than classical computers. From drug discovery to financial modeling to machine learning, quantum computers promise to revolutionize many industries. However, realizing the full potential of quantum computing requires overcoming significant challenges in controlling quantum systems and developing optimized quantum algorithms. This is where artificial intelligence (AI) comes into the picture. I will explore the growing role of AI techniques in advancing quantum computing hardware and software and bringing quantum advantage to real-world applications.
Hardware-Focused AI for Quantum Control
Controlling the fragile quantum state is one of the biggest challenges facing quantum computers. Qubits represent information using quantum properties like superposition and entanglement. However, this also makes them highly prone to errors due to noise and decoherence. AI can help optimize qubit calibration, gate sequences, error detection and quantum control to improve reliability.
Researchers at quantum computing company IonQ used neural networks to model the behavior of their trapped ion qubits and adjust control voltages to maximize qubit performance. This improved two-qubit gate fidelity by 15%, which translates to 2-3 orders of magnitude speedup for quantum algorithms. Scientists at QuEra Computing and Cambridge Quantum Computing Limited developed AI agents that can automatically tune-up quantum processors in real-time, learning the optimal control solutions by interacting with the hardware directly. This could enable self-driving quantum computers!
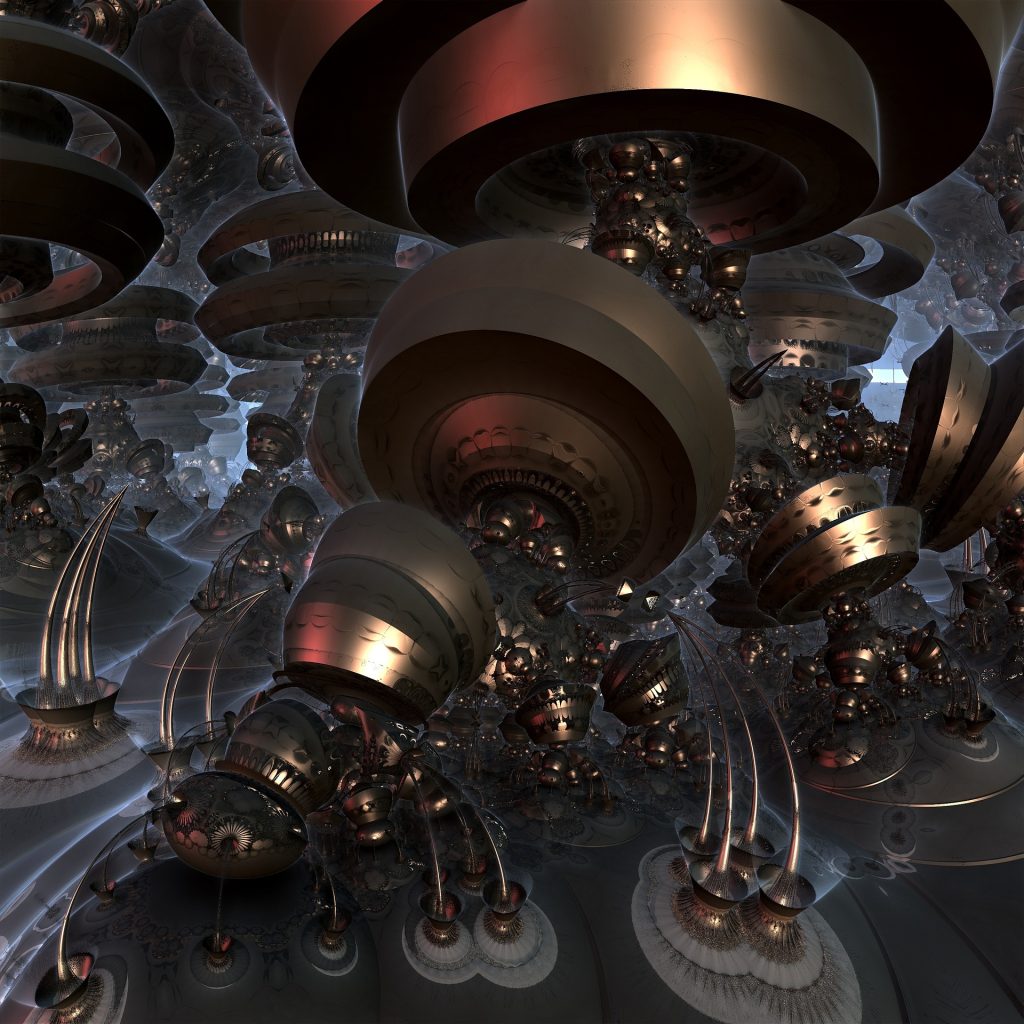
AI is also advancing novel error correction techniques tailored for quantum computers. Researchers at Google AI quantum and FermiLab designed machine learning-based error decoders that can recover quantum information from errors arising during computation. Such AI decoders lead to lower logical error rates, which will be immensely useful for scaling up quantum computers.
Software Focused AI for Quantum Algorithms
Beyond advancing quantum hardware, AI is playing an equally important role in software tools for discovering new quantum algorithms and optimizing them for real-world problems.
Researchers at quantum computing startup QC Ware created an AI blackbox tool called Qbsolv that can output optimized quantum circuits for hard combinatorial optimization problems with no knowledge of quantum mechanics principles. It works by continuously trying different permutations of gate arrangements, qubit connectivity, and control parameters to maximize the solution quality. Microsoft also developed an automated toolkit called Q# that uses reinforcement learning for discovering error-resilient implementations of quantum algorithms.
On the application front, AI techniques like quantum neural networks harness the power of quantum computing for pattern recognition and machine learning tasks like classification. Quantum machine learning promises exponential speedups compared to classical deep learning. Startup Quantinuum InQuanto leverages quantum neural networks running on quantum hardware to recognize handwritten digits with high accuracy. Researchers across IBM, Google, and Amazon are also exploring quantum machine learning for drug discovery, quantum chemistry simulation and financial risk modeling with significant results.
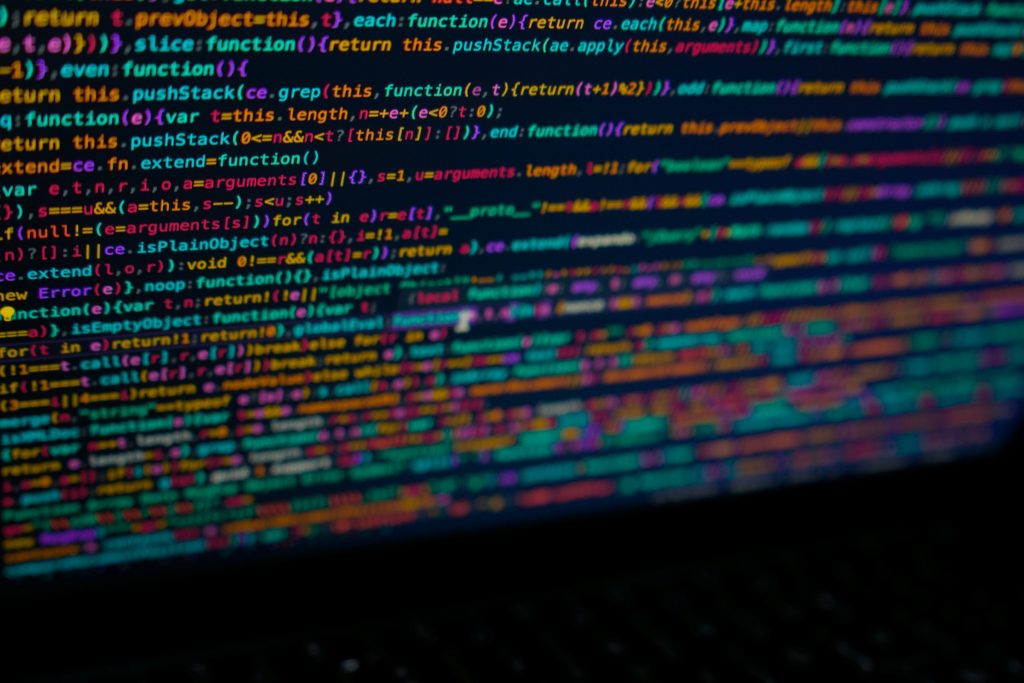
Challenges in Building Quantum-AI Hybrid Systems
While the synergy between AI and quantum computing offers new and exciting possibilities, there are considerable challenges that need to be addressed.
A key hardware challenge stems from the vastly different computing architectures followed by AI and quantum systems. The sequential von Neumann architecture of classical computers is ill-suited for control and measurement of quantum processors which require massive parallelism. This necessitates specialized system architectures for tightly integrating classical AI resources and quantum co-processors – an area requiring more research.
There are also serious software challenges that need to be tackled to build robust hybrid quantum-AI solutions. Firstly, we require optimized compilers, simulators and benchmarking tools for debugging, error-analysis and performance profiling of complex quantum-classical algorithms. Secondly, quantum and classical data encoding schemes are quite disjoint, necessitating specialized input-output interfaces for seamless data transfer between quantum and classical hardware – an area ripe for innovation using AI techniques.
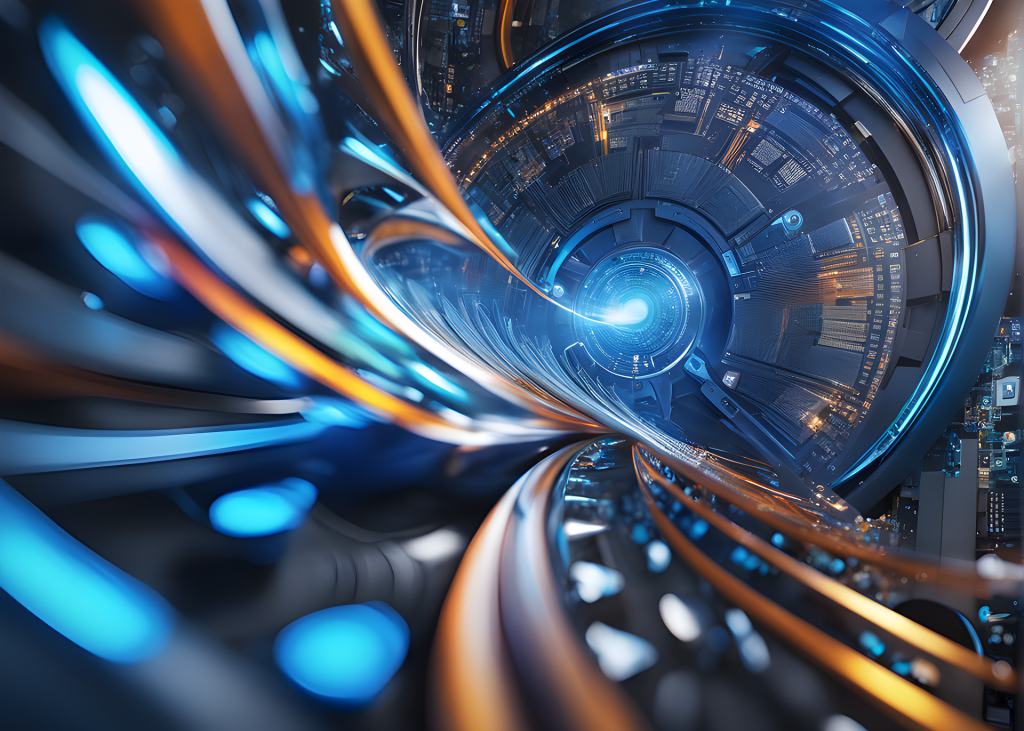
Finally, from an application perspective while quantum computing shows much promise, not all AI algorithms will see quantum advantage – we need enhanced models for characterizing the time and space complexity of AI workloads on quantum hardware. Careful analysis is crucial for determining which parts of the AI pipeline can leverage quantum speedup. Initiatives around developing such AI-quantum complexity theory combined with empirical evaluation of quantum machine learning workflows will be impactful.
The Path Forward
Incorporating the complementary strengths of AI and quantum computing is pivotal for unlocking real-world quantum advantage. National initiatives like the Quantum Economic Development Consortium (QED-C) and startups like QC-Ware, Multiverse Computing, and Riverlane are bringing together expertise across business, academia and government to build out robust quantum-AI ecosystems.
Hardware focused partnerships like those between IBM and MIT-IBM Watson AI Lab as well as Amazon Braket and Caltech aim to deeply integrate quantum and classical resources minimizing latency for hybrid quantum-AI programs. Collaboration with industry users in the finance, pharmaceutical and automotive sectors is also vital for benchmarking quantum-AI applications on real-world problems ranging from portfolio optimization to quantum chemistry.
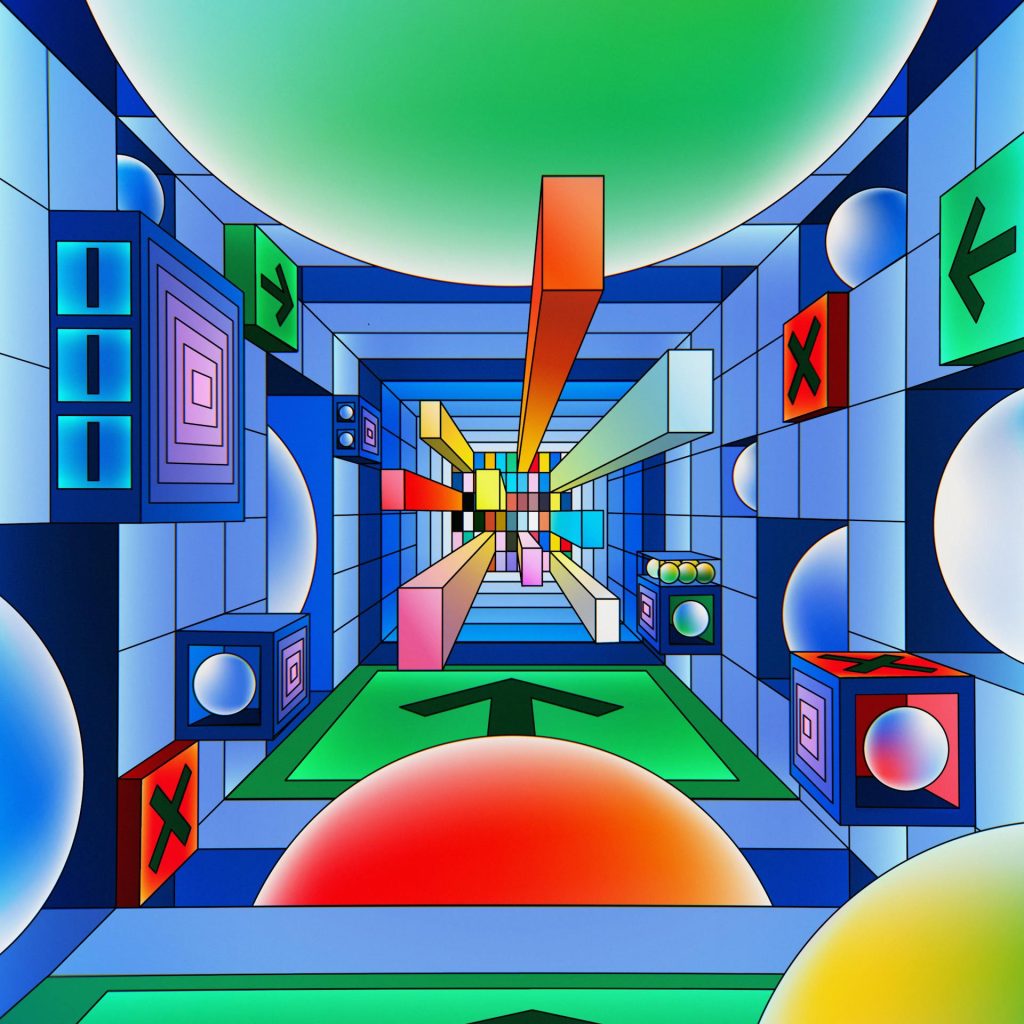
As quantum hardware and hybrid system architectures mature over the next 5 years, we will see tremendous innovation in AI techniques for quantum control, error correction and algorithm development. This will be complemented by advances in quantum machine learning and quantum neural networks leveraging computational graphs distributed across classical and quantum processors. The stage is set for AI to become a definitive enabler bridging the gap between noisy intermediate-scale quantum (NISQ) computers of today and the fault-tolerant machines of tomorrow!
Copyright©dhaka.ai
tags: Artificial Intelligence, Ai, Dhaka Ai, Ai In Bangladesh, Ai In Dhaka, USA