Deepmind revealed the launch of AlphaFold 3, the next evolution of its revolutionary AI software for structural biology. This cutting-edge technology has already enabled thousands of researchers to predict the intricate chemical interactions involved with proteins, and the new iteration promises even greater capabilities.
A Major Leap Forward One of the most significant advancements in AlphaFold 3 is its ability to predict not only protein folding but also the interactions of other crucial biological molecules, including DNA, RNA, and small molecules. Deepmind proudly published the accuracy of its predictions in the prestigious journal Nature.
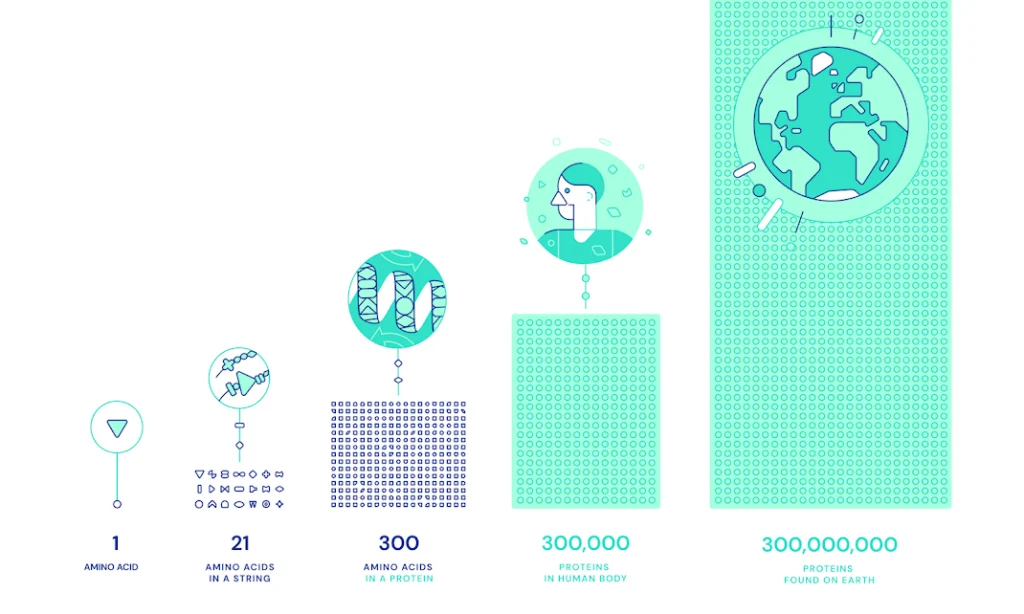
“The response to AlphaFold 2 was more than I could have ever imagined in terms of the kind of creativity and what the research community has done with it,” said John Jumper, Deepmind’s research director, at a press briefing. “And I can’t wait to see what they do with AlphaFold 3.”
Increased Accessibility In addition to its expanded capabilities, Deepmind has launched the AlphaFold Server, a web service that allows researchers to generate biochemical models without the need to install the system’s open-source code, as was required with previous iterations. This move significantly enhances the accessibility of this groundbreaking technology for the scientific community.
Another notable advancement is AlphaFold’s integration with diffusion models, which have gained popularity for their use in AI systems that generate images from text prompts. These models have now replaced some of AlphaFold’s previous structural models, further enhancing its predictive power.
The Protein-Folding Challenge To appreciate the magnitude of AlphaFold’s achievement, it’s essential to understand the “protein-folding problem” that it has addressed. Proteins, the building blocks of all organic life, comprise long chains of amino acids that fold like ‘origami’ into 3D structures that determine their functions. Understanding how these structures fold opens the door to deciphering the molecular mechanisms that underpin health and disease.
However, proteins can also become misfolded, a process that not only disrupts their normal function but also contributes to the development of diseases such as Alzheimer’s and Parkinson’s. Misfolding can interfere with cellular health by accumulating dysfunctional proteins that can damage cells and tissues.
Prior to machine learning methods, the number of possible configurations a protein can take is astronomically high, making it computationally intensive to predict the correct structure through manual calculations or brute force alone. This “protein folding problem” had long been a grand scientific challenge.
AlphaFold’s Solution AlphaFold solves the issue of scale in predicting protein structures using deep learning. The system uses neural networks trained on a database of known protein structures to infer the 3D shape of proteins from their amino acid sequences.
The recently announced AlphaFold 3 features an improved version of the Evoformer module, a key component of the deep learning architecture underpinning AlphaFold 2. Once the Evoformer module processes input molecules, AlphaFold 3 uses a novel diffusion network, similar to those used in AI image generators like DALL-E, to assemble the predicted structures. This network starts with a ‘cloud’ of atoms and iteratively refines the structure over a series of steps until it converges on a final, likely accurate molecular configuration.
A Short History of the AlphaFold Project The AlphaFold project’s origins date back to 2016, shortly after AlphaGo’s historic victory against Lee Sedol, a top international Go player. In 2018, DeepMind debuted AlphaFold 1, the first version of the AI system, at the CASP13 (Critical Assessment of Protein Structure Prediction) challenge. This biennial competition brings together research groups from around the world to test the accuracy of their protein structure predictions against real experimental data. AlphaFold 1 placed first in the competition, marking a massive milestone in computational biology.
Two years later, at CASP14 in 2020, DeepMind presented AlphaFold 2, demonstrating an accuracy so high that the scientific community considered the protein-folding problem essentially solved. AlphaFold 2 achieved a median accuracy score of 92.4 GDT (Global Distance Test) across all targets, a remarkable feat considering that a score of 90 GDT is considered competitive with results obtained from experimental methods.
The AlphaFold 2 methods paper has since received over 20,000 citations, placing it among the top 500 most-cited papers across all scientific fields. AlphaFold has been instrumental in numerous novel research projects, such as studying proteins that might degrade environmental pollutants like plastics and improving our understanding of uncommon tropical diseases like Leishmaniasis and Chagas.
Sharing the Power of AlphaFold Deepmind has been committed to sharing the widespread benefits of its AlphaFold technology with the research community. In July 2021, in partnership with EMBL’s European Bioinformatics Institute (EMBL-EBI), DeepMind released the AlphaFold Protein Structure Database, which provided access to over 350,000 protein structure predictions, including the complete human proteome.
“This will be one of the most important datasets since the mapping of the Human Genome,” said Professor Ewan Birney, EMBL Deputy Director General and EMBL-EBI Director.
This release dramatically expanded our knowledge of protein structures, more than doubling the number of high-accuracy human protein structures available to scientists.
On 28 July 2022, Deepmind expanded this database from nearly one million structures to over 200 million structures, encompassing nearly all cataloged proteins known to science. To date, the AlphaFold Protein Structure Database has been accessed by over one million users in over 190 countries, enabling discoveries in fields ranging from medicine to agriculture and beyond.
Most recently, Deepmind launched the AlphaFold Server, a free and easy-to-use research tool powered by AlphaFold 3. This platform allows biologists to generate molecular complexes with just a few clicks, regardless of their access to computational resources or their expertise in machine learning.
Accelerating Scientific Discovery The impact of AlphaFold on the scientific community has been profound. Millions of researchers globally have used AlphaFold to accelerate progress on important real-world problems, including breaking down single-use plastics, solving biological puzzles, and finding new malaria vaccines. By reducing the need for slow and expensive experiments, AlphaFold has potentially saved the research world hundreds of millions of researcher-years of progress and trillions of dollars.
A quarter of research that makes use of AlphaFold is related to understanding and tackling diseases that cause millions of deaths globally. The Drugs for Neglected Diseases initiative is advancing drug discovery for neglected diseases, such as Chagas disease and leishmaniasis, which impact millions of people, particularly within poor and vulnerable communities.
At the University of Cambridge, a team is using AlphaFold to search for a more effective malaria vaccine, while at the University of Colorado, Boulder, another team is studying antibiotic resistance – a problem that results in nearly 3 million infections in the US alone each year.
The impacts of AlphaFold are realized through how it empowers scientists to accelerate discovery across open questions in biology and new lines of research. As Deepmind’s research director, John Jumper, stated, “We’re just beginning to tap into AlphaFold’s potential, and we can’t wait to see what the future holds.”
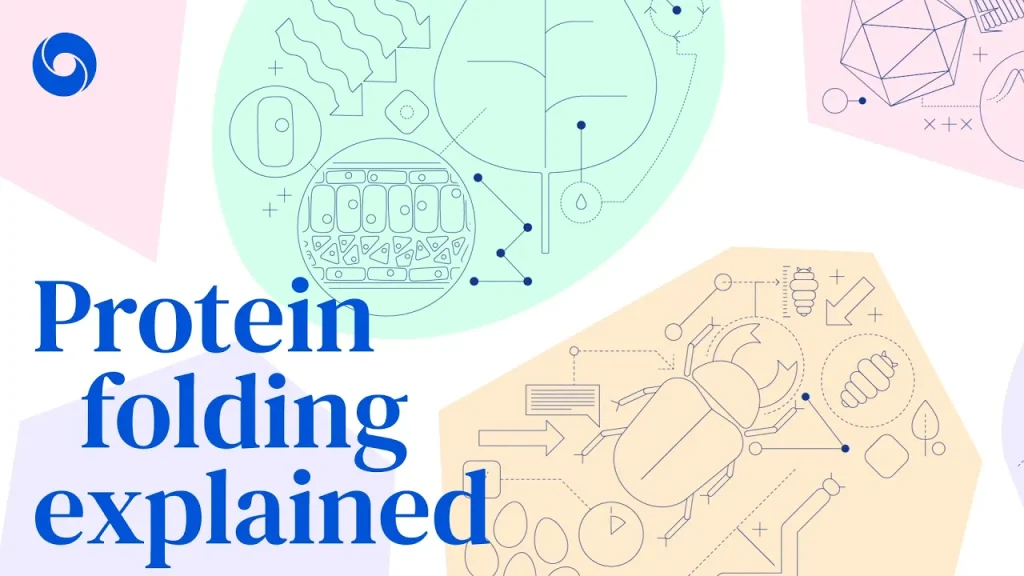
AlphaFold 3 represents a groundbreaking advancement in the field of structural biology, offering researchers unprecedented capabilities in predicting the intricate interactions of biological molecules. By solving a decades-old scientific challenge, this AI system is paving the way for crucial breakthroughs in areas such as disease treatment, environmental sustainability, and perhaps even unraveling the mysteries of life itself.
Copyright©dhaka.ai
tags: Artificial Intelligence, Ai, Dhaka Ai, Ai In Bangladesh, Ai In Dhaka, Google, Claude, Future of AI, AlphaFold3