Climate change represents the most pressing existential threat facing humanity today. As global temperatures rise, extreme weather events become more frequent, and ecosystems suffer irreparable damage, urgent and unprecedented action is imperative to mitigate climate impacts and build resilience. In this decisive battle against the climate crisis, artificial intelligence (AI) is emerging as a pivotal technology that can substantially boost decarbonization and adaptation efforts across sectors.
From predicting extreme weather with pinpoint accuracy to optimizing complex renewable energy systems, AI holds remarkable potential to accelerate our transition to a low-emissions, climate-resilient future. Real-world case studies clearly demonstrate this transformative promise:
Precision Forecasting Limits Disaster Impact
As anthropogenic climate change increases the intensity, frequency and unpredictability of natural disasters, early warning systems enabled by machine learning algorithms can give vulnerable communities vital additional hours or days to prepare.
For instance, Google utilizes artificial intelligence coupled with vast troves of geospatial data to build high-resolution flood forecasting models covering over 460 million people globally. By analyzing satellite imagery alongside complex variables like terrain characteristics, precipitation patterns and flood histories, AI systems can accurately predict impending flood timing, geographic location, inundation depth, duration and more up to 30 days in advance. Armed with such granular forecasts, disaster management agencies can issue localized warnings to prompt evacuations in high-risk areas, while strategically pre-positioning relief personnel and supplies.
During Cyclone Amphan in 2020, these AI-powered alerts allowed over 12 million coastal residents across India and Bangladesh to find safety, while targeted information empowered response teams to clear roads, stabilize infrastructure and restore communication channels before landfall. The resulting reduction in loss of life and economic damages underscores the immense value of artificial intelligence in extreme weather early warning systems.
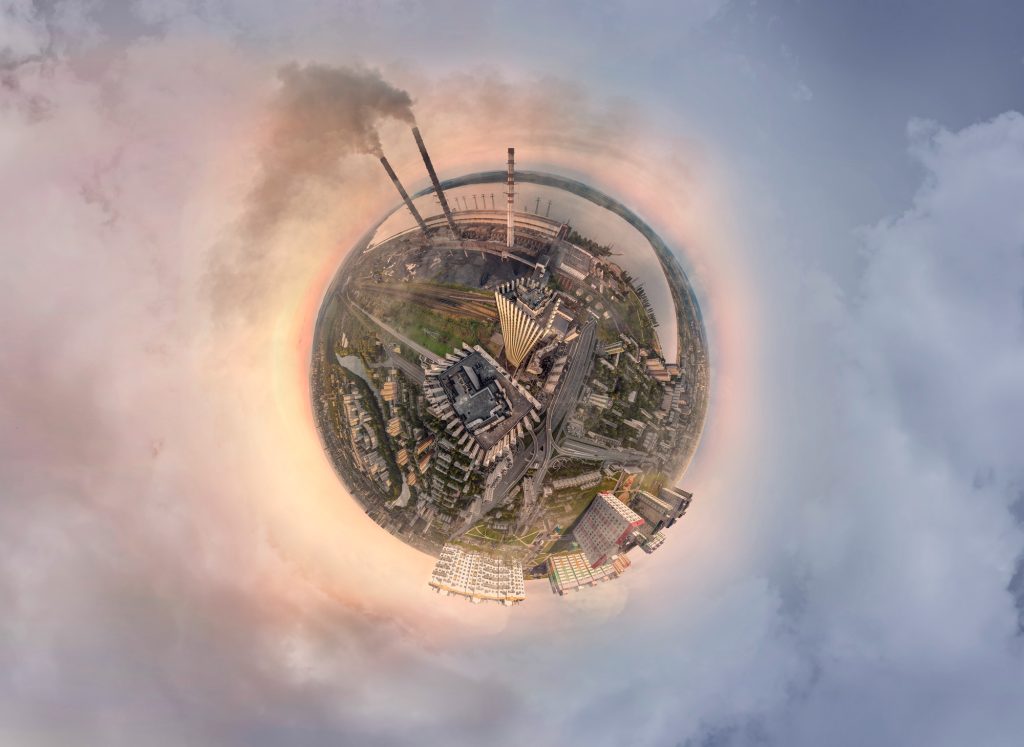
American Airlines uses similar contrail prediction technology to proactively adjust flight paths based on hyper-localized weather conditions, avoiding substantial emissions from airplane condensation trails that trap heat in the atmosphere. By understanding how altitude, atmospheric variables like humidity and temperature gradients determine contrail formation, AI algorithms can identify flight corridors to minimize contrail incidence and intensity.
Scaling Renewable Energy Adoption
The renewable energy transition represents the linchpin underpinning global decarbonization goals, with AI set to play an instrumental role in overcoming intermittency challenges associated with solar, wind and tidal generation. Sophisticated renewable energy management platforms use machine learning techniques to forecast renewable output, predict fluctuations in energy demand, balance electricity supply and demand in real-time, and optimize dynamic storage solutions to match supply with consumption. Such smart balancing enabled by AI smooths the integration of larger shares of renewables onto electricity grids while maintaining or even enhancing grid stability and reliability.
For instance, UK-based startup RatedPower provides a machine learning platform that forecasts solar energy potential based on weather data, equipment configurations and site characteristics. The technology optimizes system specifications tailored to location and budget for maximize power generation, empowering faster adoption of rooftop and large-scale solar installations.
Applied globally, such AI-enabled smart renewable grids have the potential to accommodate up to 80% renewables without destabilization. This could potentially reduce annual worldwide CO2 emissions by up to 2.1 gigatons by 2030 – equivalent to permanently taking all US passenger vehicles off the road.
Reinforcing Climate Accountability
Corporate and government transparency and accountability represent fundamental pillars of the climate response. Here too, AI solutions play a crucial role by exploiting satellite imagery and Internet of Things (IoT) sensor data to pinpoint pollution sources and independently verify official emissions reports. Startups like WattTime use computer vision algorithms trained to automatically detect visual signs of carbon emissions like smokestacks, gas flares and mine waste pools. By combining this with emission factor data, AI quantification models can rapidly estimate facility-level outputs across power plants, refineries, mines and factories around the world.
Such micro-level emissions tracking capabilities help plug information gaps, facilitate auditing of corporate climate pledges, and drive transparent climate action by empowering watchdog groups to hold major polluters financially and legally accountable.
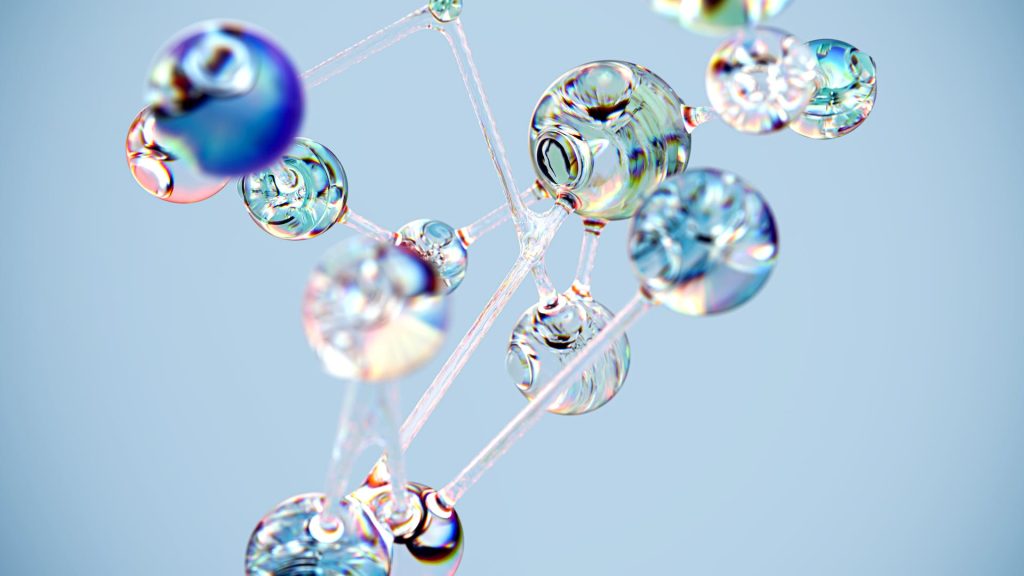
Optimizing Carbon Capture
Even as we race to curb emissions, carbon capture and sequestration technologies offer enormous potential for actively drawing down atmospheric CO2 levels. However, real-world implementation remains limited by prohibitively high costs and energy penalties. Yet again, artificial intelligence opens up an innovative solution pathway – IBM researchers are utilizing quantum computing augmented with machine learning techniques to model millions of hypothetical carbon capture solvent combinations. This enables the discovery of entirely novel materials optimized for efficiency and scalability at a fraction of the time and cost.
Microsoft AI for Earth also deploys cloud computing for climate solutions, using machine learning to analyze reams of data from field chemical sensors and lab experiments. The resulting insights help refine solvents and streamline modular carbon capture systems for factories, power plants and other decentralized applications.
Such AI-based optimization and novel material discovery can boost efficiency across the gamut of climate solutions – from predictive maintenance sensors that curb turbine downtime at wind farms to resilient coastal infrastructure designed to withstand rising sea levels.
Tailoring Adaptation Strategies
As climate change threatens health, food security, water availability and economic stability for vulnerable communities worldwide, machine learning and geospatial analytics empower decision-makers to prepare adaptive strategies tailored to localized realities. Startups like São Paulo’s SIPREMO couple high-resolution weather predictions with demographic analytics to simulate hyperlocal disaster scenarios – projecting fine-grained climate risk profiles unique to every neighborhood. These AI-powered visualizations reveal disparate impacts based on topography, urban density and socioeconomic differences, allowing municipal corporations to equitably prioritize adaptation resources to maximize community resilience.
In coastal Bangladesh, AI simulation models help policymakers identify communities most vulnerable to storm surges, salinization and changing fisheries patterns driven by rising seas. Agricultural support and livelihood retraining programs developed based on this micro-targeting can build climate resilience for those most at risk.
The Road Ahead: Opportunities and Challenges
With manifold real-world applications already reaping meaningful climate and humanitarian impact, AI’s emissions reduction potential alone is estimated between 5-10 GtCO2e by 2030 – equivalent to the annual carbon footprint of the European Union. Capitalizing on this promise, however, requires focused efforts to surmount key ethical challenges around energy consumption, algorithmic biases, job disruption fears and transparency concerns:
Energy and Emissions: From data centers to compute-intensive model training, rapidly expanding AI capabilities demand immense computing infrastructure support – with associated emissions estimated to reach around 0.5 GtCO2e by 2030. As private sector and research institutions push AI frontiers, sustainably powering the underlying cloud and quantum computing backends with renewable energy is critical to balance this rising footprint.
Bias and Inequity Risks: Algorithmic climate predictions and policy recommendations inherently build upon historical training data that reflects existing societal biases and inequities. As climate AI solutions proliferate, we must proactively address representation gaps in data collection processes and rigorously guard against disproportionate impacts – especially on already marginalized groups.
Job Displacement Apprehensions: Automating tasks like visual recognition, optimization and forecasting can disrupt jobs. While AI is poised to create new employment opportunities across sustainability-aligned sectors, proactive policy intervention is vital to support workforce transition programs and guarantee basic economic security for all.
The Black Box Concern: With modern AI model complexity rising exponentially through techniques like deep learning, even developers struggle to fully explain how algorithms arrive at specific outputs. While some lack of technical explainability is acceptable in narrow applications like object classification, this ‘black box’ concern intensifies for climate AI tools that inform impactful business and policy decisions. Rigorous standards around external algorithm audits, AI safety reviews and maintaining human oversight over consequential climate actions are instrumental to foster public and government trust.
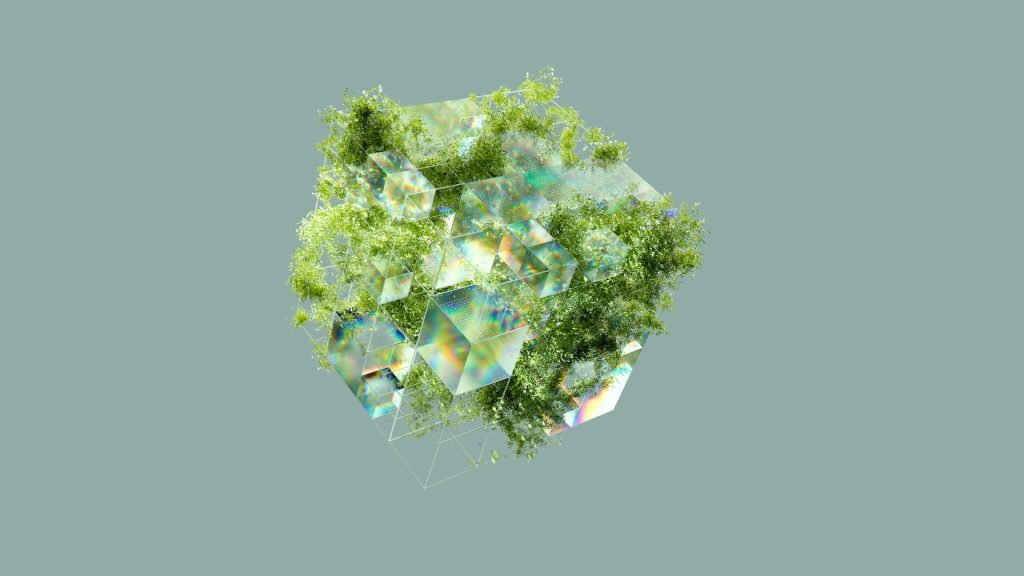
Furthermore, realizing AI’s immense potential to combat climate change requires transcending organizational silos and geographic borders to enable multi-stakeholder coordination across technology, business, policy and civil society spheres. Tech giants like Google and emerging startups both have unique strengths – the former brings vast datasets for reference models while the latter drives rapid innovation. Partnerships combining their complementary strengths can achieve more together through platforms for open knowledge exchange.
Governments play a parallel role in funding academic climate AI research, providing incentive frameworks like subsidies and procurement contracts to encourage adoption at scale, and developing forward-looking data regulations that balance privacy, security and innovation. International forums like the United Nations can also encourage technology transfer and AI capacity building to empower equitable climate progress across developed and emerging economies.
Businesses keen to tap AI’s potential must focus beyond efficiency and cost gains to prioritize environmental stewardship and climate resilience over profits. Internal training programs should nurture personnel equipped with cross-domain expertise at the intersection of sustainability and artificial intelligence to spot promising use cases.
Ultimately however, meeting the climate crisis demands both individual and collective action – from governments setting ambitious decarbonization targets and communities holding corporations accountable, to research institutions accelerating discoveries and each of us reflecting sustainable values in daily lifestyle choices.
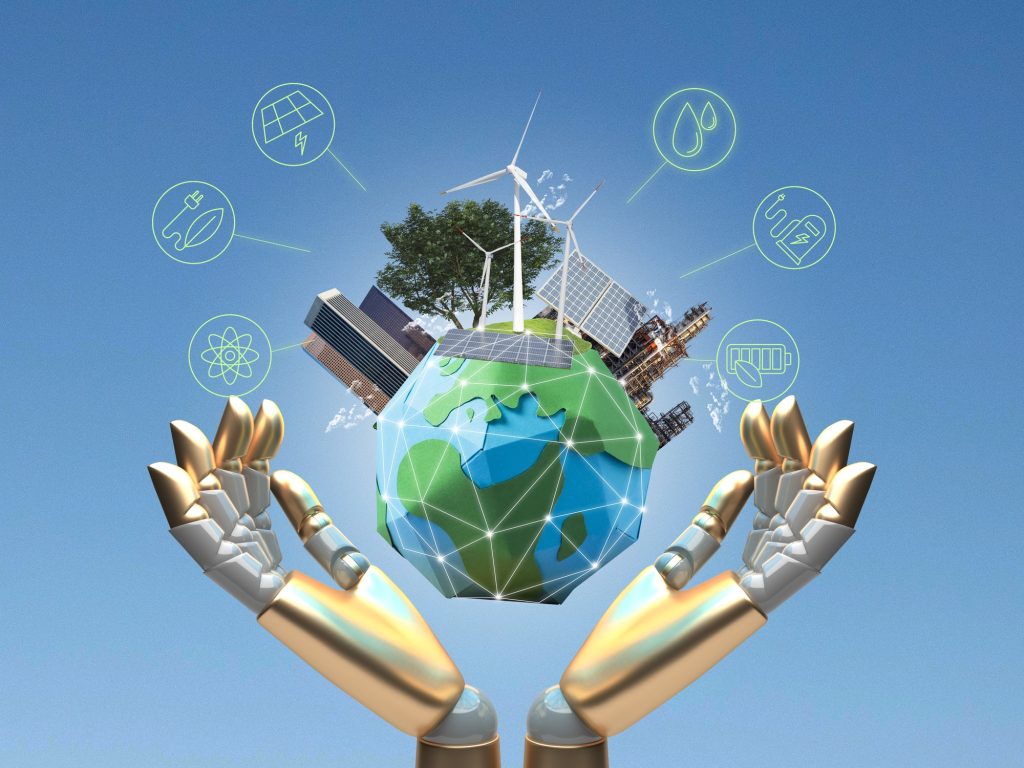
Climate change represents an all-hands-on-deck crisis demanding urgent mitigation and adaptation at unprecedented scales and speeds. In this context, artificial intelligence represents one of humanity’s most formidable digital allies with remarkable transformative potential to drive real progress. As multiplying real-world examples already demonstrate increased disaster resilience, optimized infrastructure, decentralized green technology and transparent emissions tracking
Copyright©dhaka.ai
tags: Artificial Intelligence, Ai, Dhaka Ai, Ai In Bangladesh, Ai In Dhaka, USA