Since ancient times, humans have sought to understand and anticipate the weather, a force that has shaped cultures, economies, and the very course of history. From the early Babylonian cloud watchers to the pioneering works of luminaries like Vilhelm Bjerknes and Lewis Fry Richardson, meteorology has advanced through centuries of painstaking observation and theoretical breakthroughs. However, a new revolution is underway, driven by the remarkable capabilities of artificial intelligence (AI) and machine learning (ML). This technological vanguard promises to push weather forecasting into unprecedented realms of accuracy and timeliness, with profound implications for decision-making, disaster preparedness, and our understanding of the intricate interplay between the atmosphere and human activity.
The Traditional Approach: Physics-Based Numerical Weather Prediction
For decades, the foundation of modern weather forecasting has rested upon a framework known as Numerical Weather Prediction (NWP). This approach involves translating the fundamental laws of physics that govern atmospheric behavior—principles such as fluid dynamics, thermodynamics, and radiative transfer—into complex mathematical equations. Fueled by observational data gathered from a global network of weather stations, satellites, and other sensors, these equations are then solved iteratively using powerful supercomputers to generate forecasts.
The triumph of NWP has been nothing short of remarkable, enabling meteorologists to predict weather patterns with increasing accuracy and lead times. However, this traditional method faces inherent limitations. Firstly, the sheer complexity of the equations involved and the chaotic nature of atmospheric processes can amplify even the slightest errors in initial conditions or computational approximations, leading to rapidly diverging forecast trajectories. Secondly, the computational demands of running these simulations at high resolutions and over extended periods are staggering, necessitating access to the world’s most advanced supercomputing facilities.
Moreover, NWP models struggle to capture certain phenomena, such as the intricate microphysics of cloud formation, the dynamics of severe weather events, and the intricate interplay between the atmosphere and other components of the Earth system, like the oceans and biosphere. These limitations have spurred a relentless pursuit of more advanced models, higher-resolution data, and ever-increasing computational power—a quest that, while yielding consistent improvements, has begun to approach the boundaries of feasibility and diminishing returns.
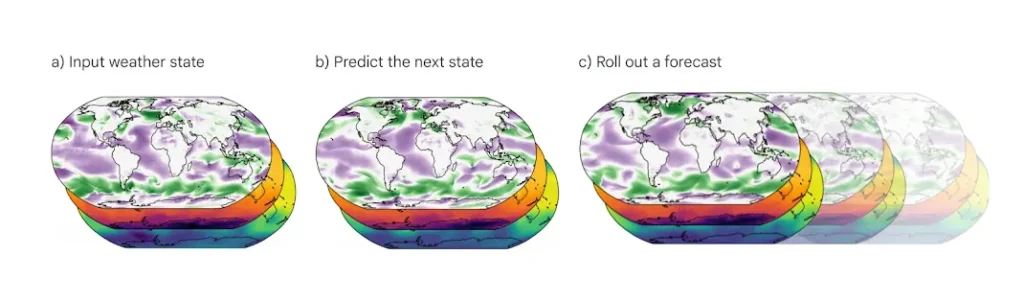
The AI Revolution: Learning from the Skies
Into this arena, AI and ML have emerged as a disruptive force, offering a fundamentally different approach to weather forecasting. Rather than relying on explicit physics-based equations, these techniques leverage the power of data and statistical learning to discern patterns and relationships within the vast troves of historical meteorological data. By ingesting decades’ worth of observational records, reanalysis datasets, and model simulations, AI systems can learn to map the complex, high-dimensional trajectories of atmospheric evolution, effectively constructing their own “equations” through the process of training.
The potential advantages of this data-driven paradigm are manifold. Firstly, AI models can bypass many of the computational bottlenecks that have constrained traditional NWP, enabling forecasts to be generated orders of magnitude faster and with a fraction of the energy consumption. Secondly, by learning directly from data, these models can potentially capture subtle patterns and nonlinear relationships that have eluded explicit mathematical formulations, potentially enhancing forecast skill for notoriously challenging phenomena like severe weather events and long-range climate projections.
Recent breakthroughs have demonstrated the transformative potential of AI in weather forecasting. In 2023, researchers at Google DeepMind unveiled GraphCast, a state-of-the-art AI model that can generate global weather forecasts up to 10 days in advance with unprecedented accuracy, outperforming the industry-standard ECMWF model on over 90% of test metrics. Even more remarkably, GraphCast can produce these forecasts in under a minute on a single desktop computer, a feat that would take conventional NWP systems hours on vast supercomputing clusters.
GraphCast’s superiority extends beyond sheer predictive accuracy; the model also demonstrated an enhanced ability to forecast severe weather events, such as tropical cyclones, atmospheric rivers, and extreme temperature anomalies. This capability could prove invaluable in mitigating the impacts of climate change-driven extreme weather, providing earlier warnings and enabling more effective disaster response planning.
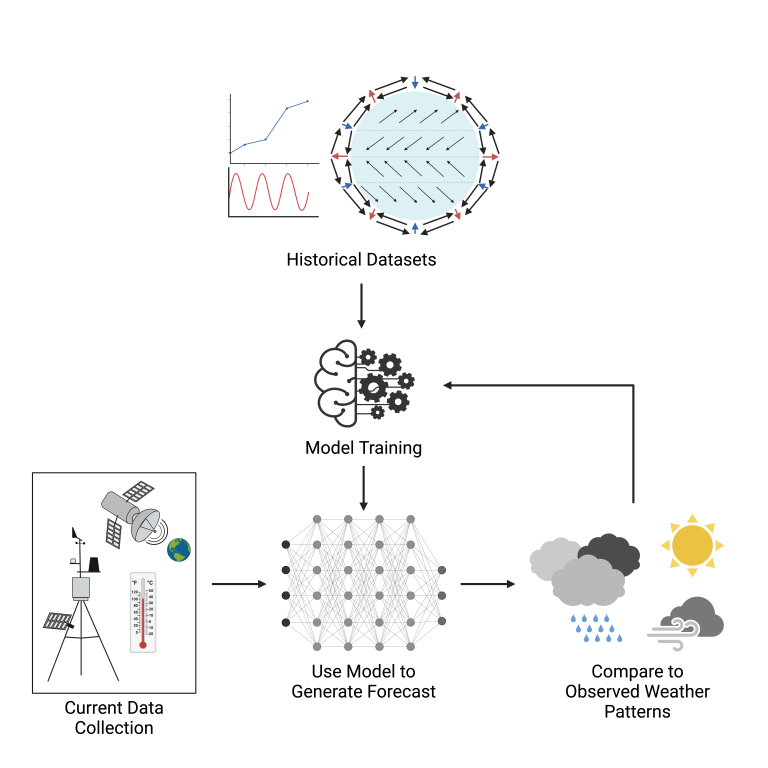
Hybrid Approaches: Combining the Best of Both Worlds
While the promise of AI-driven weather forecasting is alluring, it would be premature to declare the obsolescence of traditional physics-based models. After all, these models encapsulate centuries of scientific understanding and have been rigorously tested and refined across innumerable real-world scenarios. Moreover, the training data upon which AI models rely is itself derived, at least in part, from the output of NWP simulations, suggesting an inherent dependence on the underlying physics.
As such, many experts envision a future in which AI and traditional NWP coexist in a synergistic hybrid framework. One such approach, pioneered by researchers at Google, involves coupling a traditional “dynamical core” that simulates the broad-scale atmospheric flow with a deep learning module that captures the finer-scale physics, such as cloud microphysics and radiative transfer. This hybrid model effectively combines the strengths of both paradigms, leveraging the physical consistency and interpretability of NWP while harnessing the pattern-recognition prowess of AI to enhance forecast accuracy and computational efficiency.
Challenges and Considerations
While the potential of AI-infused weather forecasting is undeniable, several challenges and considerations must be addressed as this technology matures and becomes increasingly integrated into operational meteorology.
Interpretability and Uncertainty Quantification One of the long-standing critiques of deep learning models is their tendency to function as “black boxes,” making it challenging to understand the reasoning behind their predictions or to diagnose errors. In the context of weather forecasting, where life-or-death decisions may hinge on forecast accuracy, this lack of interpretability could be a significant barrier to adoption. Ongoing research efforts are aimed at developing more transparent and explainable AI architectures, as well as techniques for quantifying and communicating forecast uncertainty.
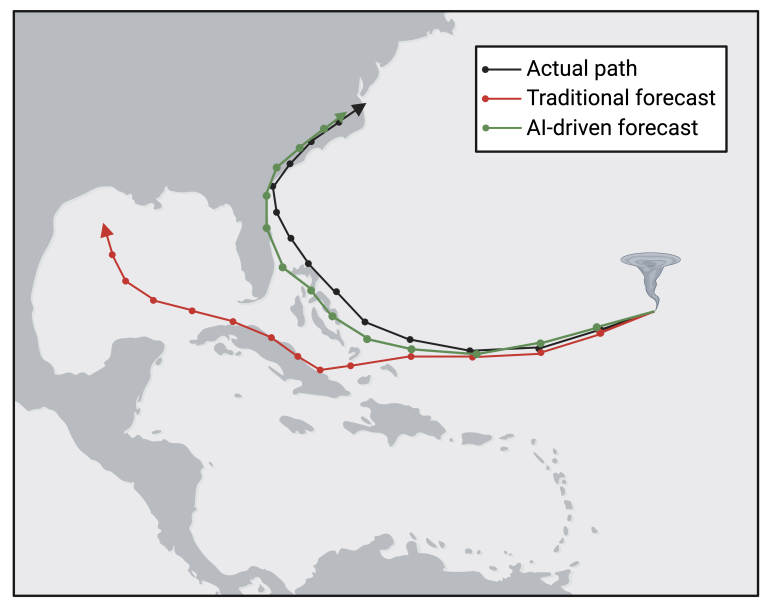
Data Quality and Bias AI models, like their human counterparts, are only as good as the data they are trained on. Inconsistencies, biases, or gaps in historical weather data could potentially propagate into the learned models, leading to systematic errors or blind spots in certain regions or weather regimes. Addressing these data quality issues, as well as accounting for the non-stationarity introduced by a changing climate, will be crucial for ensuring the long-term reliability of AI-based forecasts.
Computational Resources and Accessibility While AI models may ultimately prove more computationally efficient than traditional NWP during the forecasting phase, the training process itself can be enormously resource-intensive, often requiring vast datasets, specialized hardware (e.g., GPUs or TPUs), and significant energy consumption. As private companies like Google, Microsoft, and Huawei continue to invest heavily in this domain, concerns have been raised about the potential centralization of weather forecasting capabilities and the equitable access to these technologies, particularly for developing nations and under-resourced communities.
Ethical and Governance Considerations
The integration of AI into weather forecasting also raises broader ethical and governance questions. As private entities increasingly contribute to what has historically been a public good, mechanisms must be established to ensure transparency, accountability, and the alignment of these technologies with societal values and priorities. Issues of data privacy, algorithmic bias, and the potential for AI systems to be co-opted for nefarious purposes (e.g., weaponized weather modification) will require careful consideration and robust governance frameworks.
The Road Ahead: Embracing the AI-Driven Future of Meteorology
Despite the challenges, the integration of AI and ML into weather forecasting appears inevitable, driven by the relentless pursuit of improved accuracy, efficiency, and our collective need to better understand and adapt to a rapidly changing climate. As this technological revolution unfolds, it is crucial that the broader scientific community, policymakers, and the public remain engaged and informed, shaping the development and deployment of these powerful tools in a responsible and equitable manner.]
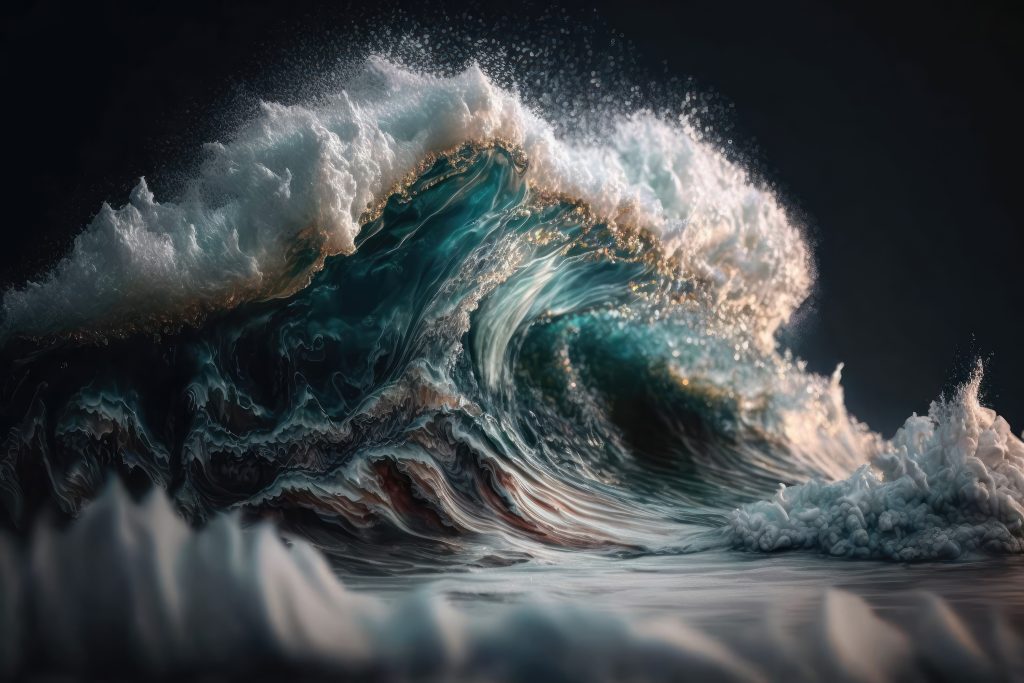
Ultimately, the true potential of AI-enhanced weather forecasting may extend far beyond mere prediction. By leveraging the ability of these models to assimilate and synthesize vast streams of environmental data, they could catalyze a deeper understanding of the intricate feedback loops and teleconnections that govern the Earth’s climate system. This knowledge, in turn, could inform more robust adaptation strategies, guide the development of resilient infrastructure, and potentially even shape interventions to mitigate the worst impacts of climate change.
In this sense, the AI revolution in meteorology represents not just a technological leap but a profound shift in our relationship with the natural world. By harnessing the power of data and machine intelligence, we may finally unlock the secrets of the skies, ushering in a new era of environmental stewardship and a deeper appreciation for the delicate balance that sustains life on our remarkable planet.
Looking ahead, the fusion of AI with weather and climate science will likely accelerate. Emerging AI technologies like large language models, capable of ingesting and reasoning over vast textual knowledge, may help bridge the gap between data-driven forecasting and the rich theoretical foundations of atmospheric science. Imagine an AI assistant that can not only generate state-of-the-art numerical predictions but also explain the underlying physical processes, draw insights from related scientific literature, and engage in substantive discourse with human experts.
Moreover, the intersection of AI and remote sensing technologies promises to revolutionize the observation and monitoring of the Earth system. Advances in machine vision, pattern recognition, and data fusion could enable more accurate and higher-resolution retrieval of atmospheric variables from satellite imagery, radar, and other sensor data. This enhanced observational capacity, coupled with AI’s ability to assimilate and interpolate sparse data, could lead to more precise initial conditions for forecast models and a deeper understanding of atmospheric dynamics across scales.
As the field progresses, we may also witness the emergence of AI-enabled decision support systems that can seamlessly integrate weather and climate forecasts with other contextual data streams, such as transportation networks, energy grids, and demographic information. These systems could provide tailored, actionable insights to stakeholders across sectors, enabling more informed and proactive decision-making in response to meteorological events.
Furthermore, the AI revolution in meteorology has the potential to catalyze a democratization of weather knowledge. By leveraging cloud computing and open-source software frameworks, powerful forecasting tools could become accessible to a broader range of users, from citizen scientists and community organizations to small businesses and local governments. This diffusion of forecasting capabilities could foster greater public engagement with weather and climate issues, empowering communities to better prepare for and respond to environmental challenges.
However, as with any disruptive technology, the integration of AI into meteorology will bring its own set of challenges and ethical considerations. Issues of data privacy, algorithmic bias, and the potential for AI systems to be co-opted for nefarious purposes (e.g., weaponized weather modification) will require careful consideration and robust governance frameworks. There is also a risk that the centralization of weather forecasting capabilities in the hands of a few powerful tech companies could exacerbate existing inequalities, with potentially dire consequences for vulnerable populations.
As such, it is imperative that the development and deployment of AI in meteorology be guided by principles of transparency, accountability, and ethical governance. Interdisciplinary collaboration between meteorologists, computer scientists, ethicists, and policymakers will be essential to ensure that these technologies are leveraged in a responsible and equitable manner, aligning with societal values and priorities.
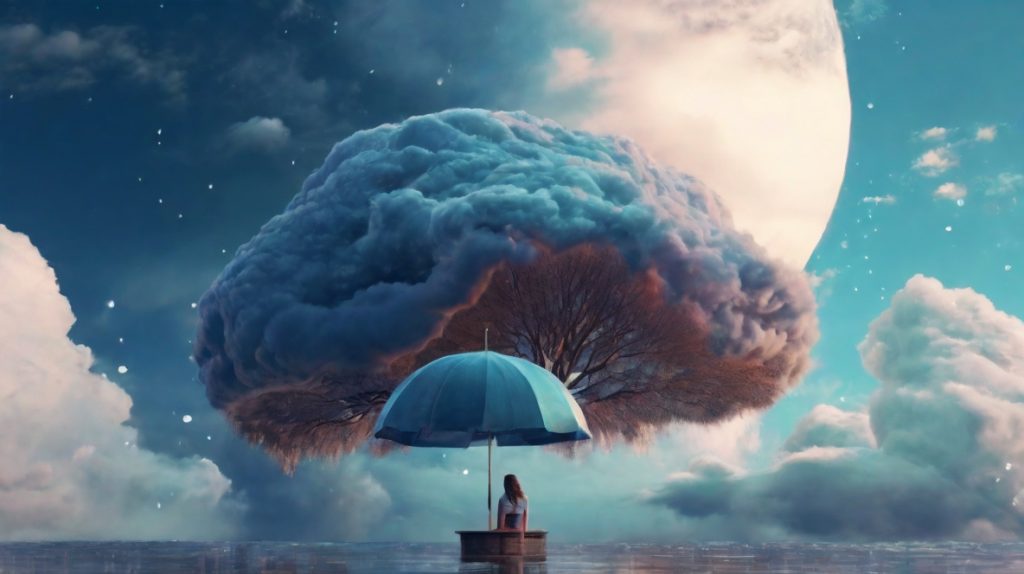
The AI revolution in weather forecasting represents a paradigm shift that transcends mere technological advancement. It is a catalyst for a deeper understanding of our planet’s intricate atmospheric processes, a harbinger of more informed and resilient decision-making in the face of environmental challenges, and a call for a reexamination of our relationship with the natural world. As we navigate this uncharted terrain, it is essential that we approach it with a sense of wonder, humility, and a steadfast commitment to using these powerful tools for the betterment of humanity and the preservation of our shared planetary home.
Copyright©dhaka.ai
tags: Artificial Intelligence, Ai, Dhaka Ai, Ai In Bangladesh, Ai In Dhaka, Google, Claude, Future of AI, Weather