In a surprising turn of events, the 2024 Nobel Prize in Physics has been awarded to John J. Hopfield and Geoffrey E. Hinton for their groundbreaking work in artificial intelligence (AI) and machine learning. This decision by the Royal Swedish Academy of Sciences marks a significant shift in recognizing the profound impact of AI research on our understanding of complex systems and computational capabilities. As we delve into the implications of this award, we’ll explore the foundational aspects of their work, its connection to physics, the current landscape of AI research, and the potential future directions of this rapidly evolving field.
The Significance of Their Work
At the heart of Hopfield and Hinton’s contributions lies the development of neural networks, which have become the backbone of modern AI technologies. Their research in the 1980s laid the groundwork for the machine learning revolution we’re witnessing today.
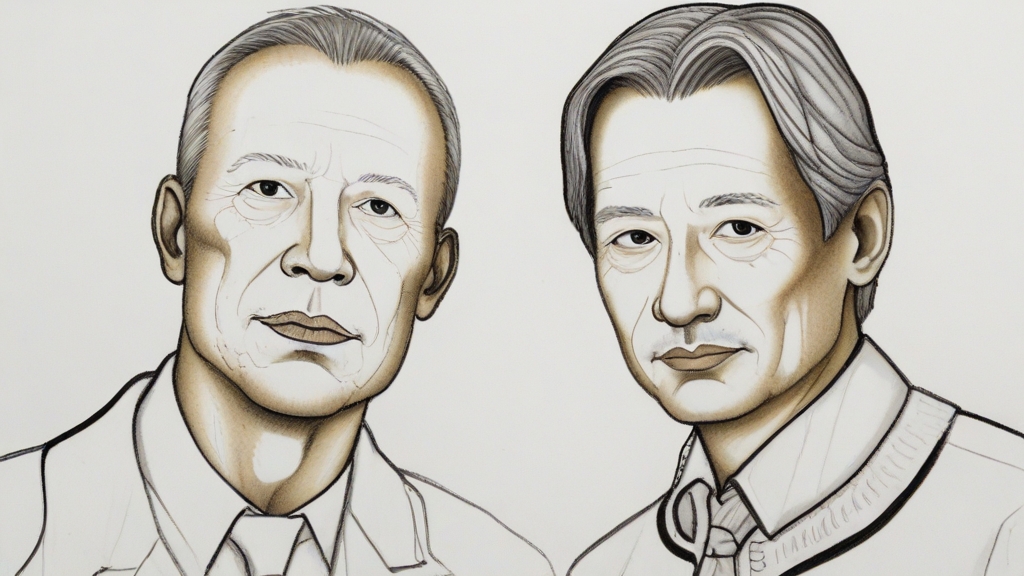
John Hopfield, a professor emeritus at Princeton University, introduced the concept of the Hopfield network in 1982. This type of artificial neural network is characterized by its ability to store and reconstruct patterns, mimicking the associative memory of biological systems. Hopfield’s work demonstrated how a network of interconnected neurons could exhibit emergent behavior, storing and retrieving information in a manner reminiscent of human memory.
Geoffrey Hinton, often referred to as a “godfather of AI,” expanded on these ideas with the introduction of the Boltzmann machine in 1985. This more complex neural network incorporated the concept of “hidden units” – additional layers of neurons between the input and output layers. This innovation allowed for more sophisticated pattern recognition and laid the foundation for deep learning, which powers many of today’s AI applications.
The significance of their work cannot be overstated. By creating these foundational models, Hopfield and Hinton provided the conceptual framework that has enabled the development of increasingly powerful AI systems. From image and speech recognition to natural language processing and generative AI, the neural network architectures they pioneered continue to be refined and expanded upon in contemporary research.
The Connection to Physics
What makes this Nobel Prize in Physics particularly intriguing is the interdisciplinary nature of Hopfield and Hinton’s work. While their research is primarily associated with computer science and AI, its roots are deeply embedded in physics concepts.
Hopfield’s approach drew inspiration from the physics of spin glasses – materials with disordered magnetic properties that exhibit complex interactions. By applying principles from statistical mechanics to artificial neural networks, Hopfield demonstrated how collective computational properties could emerge from simple, interconnected elements.
Hinton and his colleagues similarly leveraged concepts from statistical mechanics in developing the Boltzmann machine. Named after Ludwig Boltzmann, a pioneer in statistical physics, this neural network model used probabilistic rules derived from thermodynamics to govern the behavior of its nodes. This connection to physics provided a robust theoretical foundation for understanding how learning could occur in complex systems.
The Nobel Committee’s decision to award the physics prize for this work underscores the increasingly blurred lines between traditional scientific disciplines. It recognizes that fundamental concepts from physics – such as emergent behavior, statistical mechanics, and complex systems – can provide powerful tools for understanding and modeling cognitive processes and artificial intelligence.
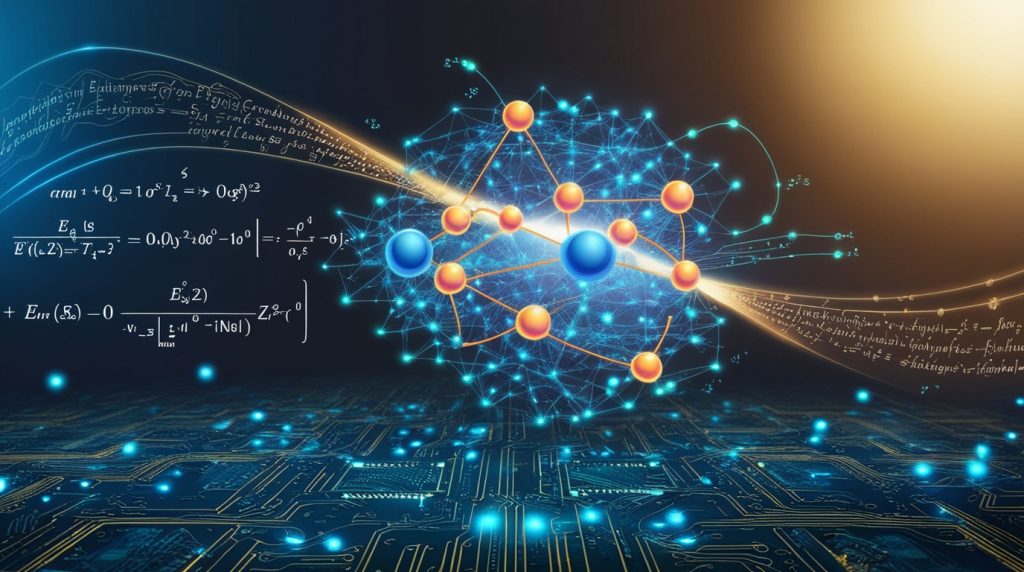
Implications for AI and Ethical Considerations
As AI technologies continue to advance at a rapid pace, the implications of Hopfield and Hinton’s work become increasingly profound. The neural network architectures they developed have evolved into the deep learning models that power everything from smartphone assistants to autonomous vehicles.
However, this progress is not without its concerns. Hinton himself has become a vocal critic of the potential dangers posed by advanced AI systems. His decision to leave Google in 2023 to speak more freely about these risks highlights the growing unease within the AI research community about the trajectory of the technology.
The primary concern is the potential for AI to surpass human intelligence in ways that could lead to loss of control or unintended consequences. As Hinton noted in his Nobel acceptance speech, “We have no experience of what it’s like to have things smarter than us.” This unprecedented situation raises a host of ethical and practical questions:
- How can we ensure AI systems remain aligned with human values and interests?
- What safeguards need to be in place to prevent the misuse of increasingly powerful AI technologies?
- How do we address the potential economic and social disruptions caused by widespread AI adoption?
- What are the implications for privacy and personal autonomy in a world of pervasive AI systems?
These questions underscore the need for ongoing dialogue between researchers, policymakers, and the public to develop ethical frameworks and governance structures for AI development and deployment.
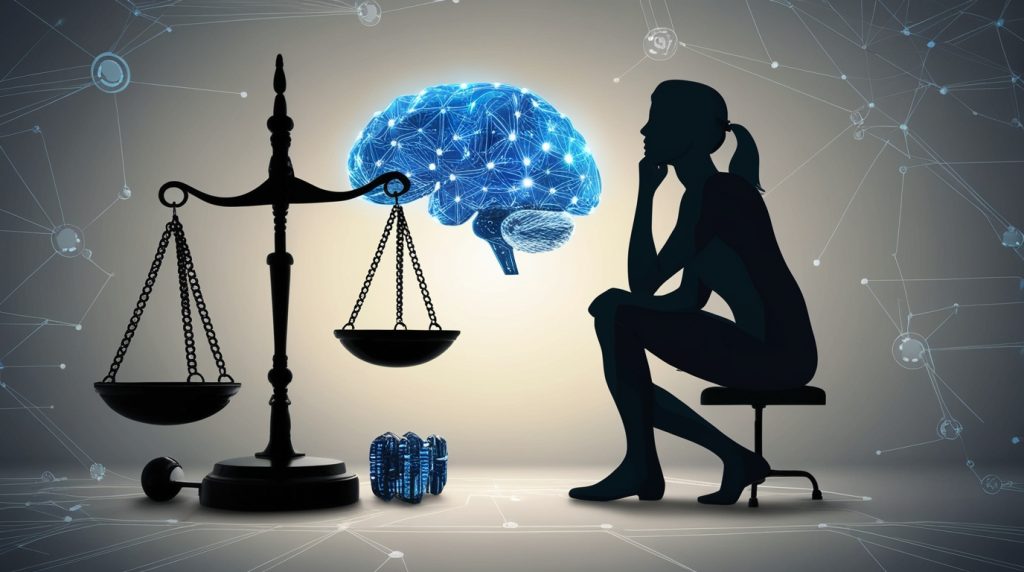
The Current Landscape of AI Research
The awarding of the Nobel Prize to Hopfield and Hinton comes at a time of unprecedented activity and progress in AI research. Building on their foundational work, researchers have developed increasingly sophisticated neural network architectures and training methodologies.
Recent years have seen remarkable advancements in areas such as:
- Natural Language Processing: Large language models like GPT-3 and its successors have demonstrated an impressive ability to generate human-like text and engage in complex language tasks.
- Computer Vision: AI systems can now recognize and analyze visual information with superhuman accuracy in many domains, from medical imaging to autonomous navigation.
- Reinforcement Learning: AI agents have mastered complex games and are being applied to real-world optimization problems in fields like energy management and logistics.
- Generative AI: Models like DALL-E and Stable Diffusion can create original images from text descriptions, pushing the boundaries of machine creativity.
- Multimodal AI: Systems that can integrate and process multiple types of data (text, images, audio) are becoming increasingly sophisticated.
These advancements have been facilitated by increases in computational power, the availability of vast datasets, and refinements to the neural network architectures first proposed by Hopfield, Hinton, and their contemporaries.
However, the field is not without its challenges and ongoing debates. Issues such as AI bias, interpretability of complex models, and the environmental impact of training large AI systems are at the forefront of current research efforts. Additionally, there is ongoing discussion about the best approaches to achieving artificial general intelligence (AGI) – systems with human-level cognitive abilities across a wide range of tasks.
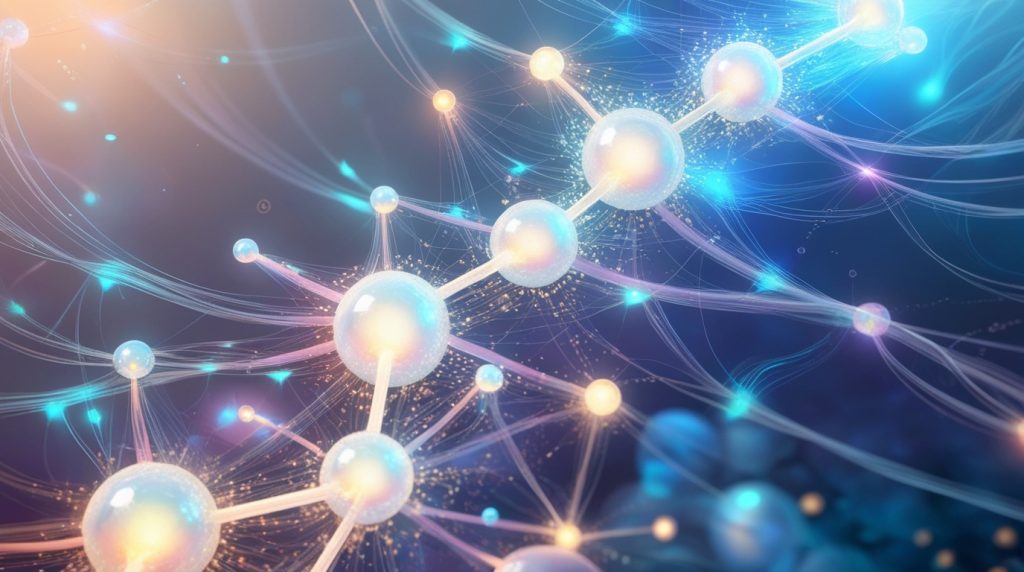
Impact on Society
The influence of AI technologies derived from Hopfield and Hinton’s work is being felt across virtually every sector of society. Some key areas of impact include:
- Healthcare: AI is revolutionizing medical diagnosis, drug discovery, and personalized treatment planning. Machine learning models can analyze medical images with high accuracy and identify patterns in patient data that might elude human physicians.
- Scientific Research: AI is accelerating scientific discovery in fields ranging from astrophysics to materials science. Machine learning models can process vast datasets, identify promising research directions, and even generate hypotheses for further investigation.
- Engineering and Design: AI-powered tools are enhancing the capabilities of engineers and designers, enabling rapid prototyping and optimization of complex systems.
- Education: Adaptive learning systems powered by AI are personalizing education, providing tailored instruction and feedback to students based on their individual needs and progress.
- Finance: AI algorithms are being used for fraud detection, risk assessment, and algorithmic trading, transforming the financial services industry.
- Environmental Protection: Machine learning models are helping to monitor and predict environmental changes, optimize resource usage, and develop more sustainable technologies.
While these applications offer tremendous potential benefits, they also raise important societal questions. The increasing reliance on AI systems for decision-making in critical areas such as healthcare, criminal justice, and financial services necessitates careful consideration of issues like accountability, transparency, and fairness.
Moreover, the potential for AI to automate many existing jobs has significant implications for the future of work and economic structures. Ensuring that the benefits of AI are broadly distributed and that workers are prepared for a changing job market will be crucial challenges in the coming years.
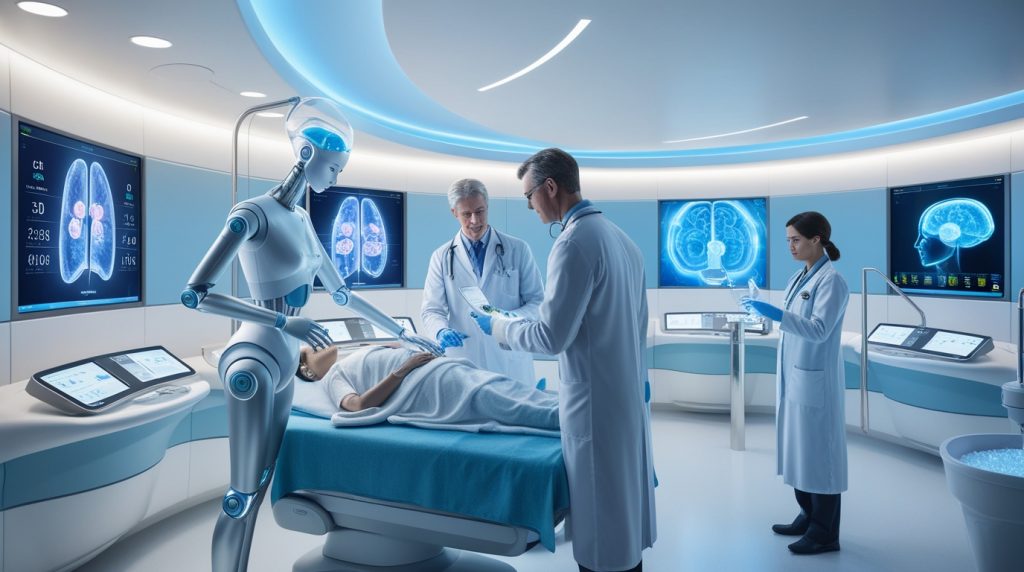
Future Directions
As we look to the future, the work of Hopfield and Hinton continues to influence the trajectory of AI research and development. Several key trends and areas of focus are likely to shape the field in the coming years:
- Improved Efficiency: Researchers are working on developing more energy-efficient AI models and training methods to address the environmental concerns associated with large-scale AI systems.
- Enhanced Interpretability: There is a growing focus on creating AI systems that can explain their decision-making processes, which is crucial for building trust and enabling deployment in sensitive applications.
- Robust and Reliable AI: Developing AI systems that are resilient to adversarial attacks and can perform consistently across diverse environments remains a key challenge.
- Ethical AI: The development of AI systems that can reason about ethical considerations and make decisions aligned with human values is an area of increasing importance.
- Brain-Inspired Computing: Continued exploration of how biological neural networks function could lead to new paradigms in AI architecture and computation.
- Quantum AI: The intersection of quantum computing and AI holds promise for solving certain classes of problems exponentially faster than classical systems.
- Human-AI Collaboration: Rather than viewing AI as a replacement for human intelligence, there is growing interest in developing systems that can effectively augment and collaborate with human capabilities.
As these areas of research progress, it will be crucial to continue the interdisciplinary approach exemplified by Hopfield and Hinton’s work. The complex challenges posed by advanced AI systems will require insights from computer science, neuroscience, physics, philosophy, and social sciences.
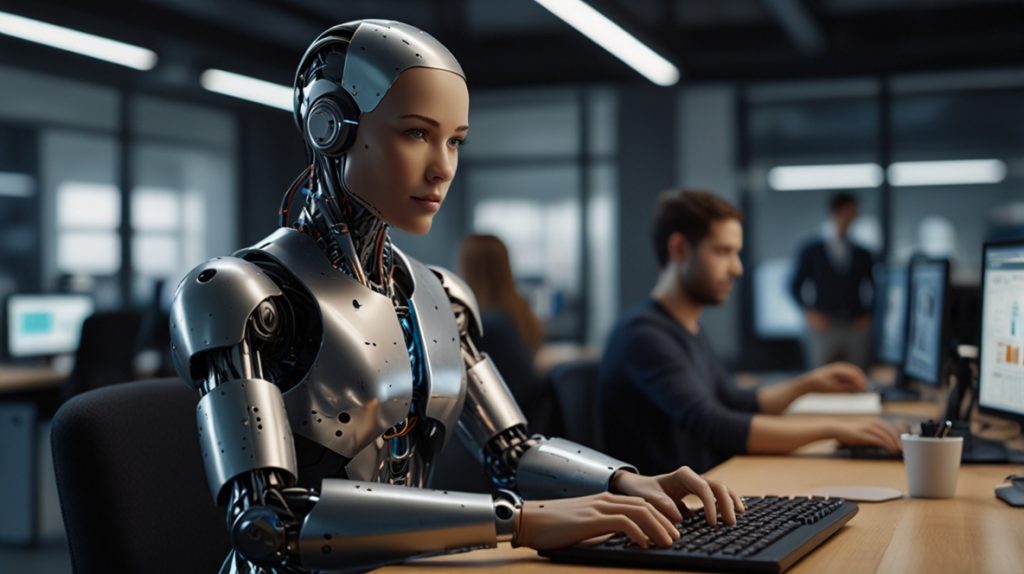
The awarding of the 2024 Nobel Prize in Physics to John Hopfield and Geoffrey Hinton marks a pivotal moment in the recognition of AI research as a fundamental scientific endeavor. Their work, bridging concepts from physics and computer science, has laid the foundation for a technological revolution that is reshaping our world.
As we celebrate their achievements, we must also grapple with the profound implications of the technologies they helped create. The potential benefits of AI in areas like healthcare, scientific discovery, and environmental protection are immense. However, the risks and ethical challenges posed by increasingly powerful AI systems cannot be ignored.
Moving forward, it will be essential to foster a collaborative approach to AI development that brings together researchers, policymakers, and the public. By doing so, we can work towards harnessing the power of AI to address global challenges while ensuring that these technologies remain aligned with human values and interests.
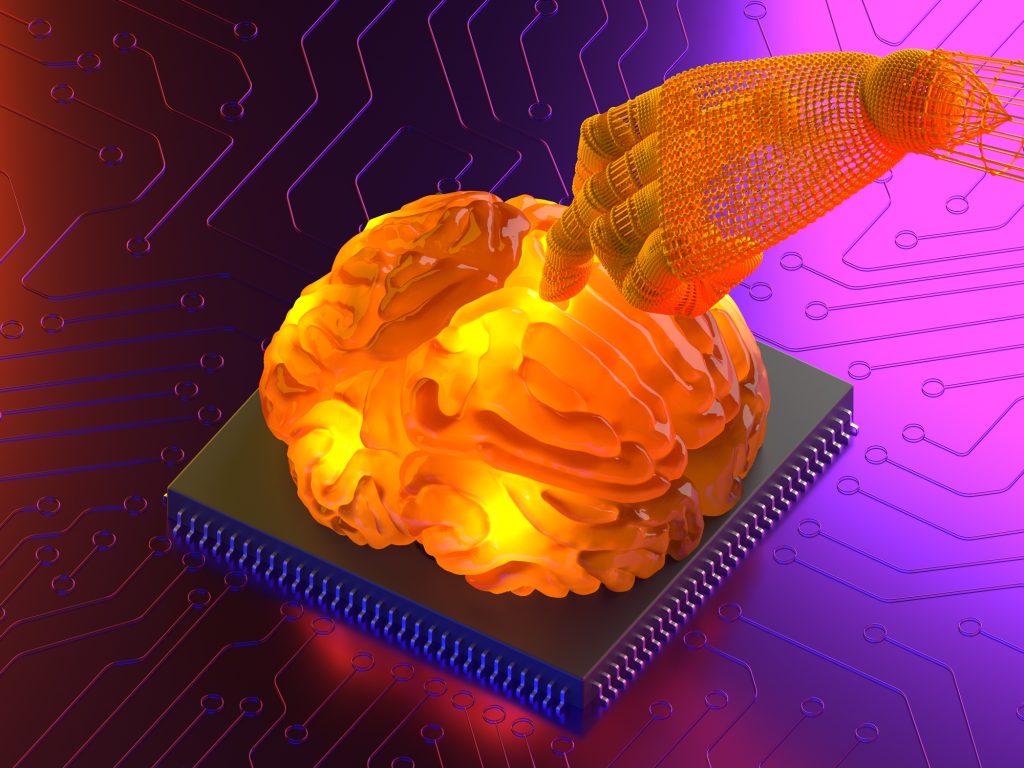
The story of Hopfield and Hinton’s Nobel Prize is not just about recognizing past achievements; it’s a call to action for shaping the future of AI in a way that benefits all of humanity. As we stand on the threshold of a new era of intelligent machines, their work reminds us of the power of interdisciplinary thinking and the importance of considering the long-term implications of our technological innovations.
Copyright©dhaka.ai
tags: Artificial Intelligence, Ai, Dhaka Ai, Ai In Bangladesh, Ai In Dhaka, Future of AI, Artificial Intelligence in Bangladesh, Nobel Prize 2024