In a world where artificial intelligence is often equated with immense complexity and daunting computational resources, Microsoft has taken an unconventional path – one inspired by the simplicity of bedtime stories. The tech giant has unveiled the Phi-3 family of open small language models (SLMs), a groundbreaking development that promises to make AI more accessible and cost-effective for a broader range of users and applications.
At the heart of this innovation lies a surprising insight that emerged from a moment of serendipity. As Ronen Eldan, a Microsoft Research machine learning expert, read bedtime stories to his daughter, he found himself pondering the remarkable ability of children to grasp language. “How did she learn this word? How does she know how to connect these words?” he wondered, according to a Microsoft blog post. This seemingly innocuous question sparked a profound realization: perhaps the key to unlocking the potential of small language models lay in embracing the simplicity of a child’s vocabulary and understanding.
The result of this epiphany is a series of compact yet remarkably capable SLMs that defy conventional wisdom. “Sometimes the best way to solve a complex problem is to take a page from a children’s book. That’s the lesson Microsoft researchers learned by figuring out how to pack more punch into a much smaller package,” the blog post states.
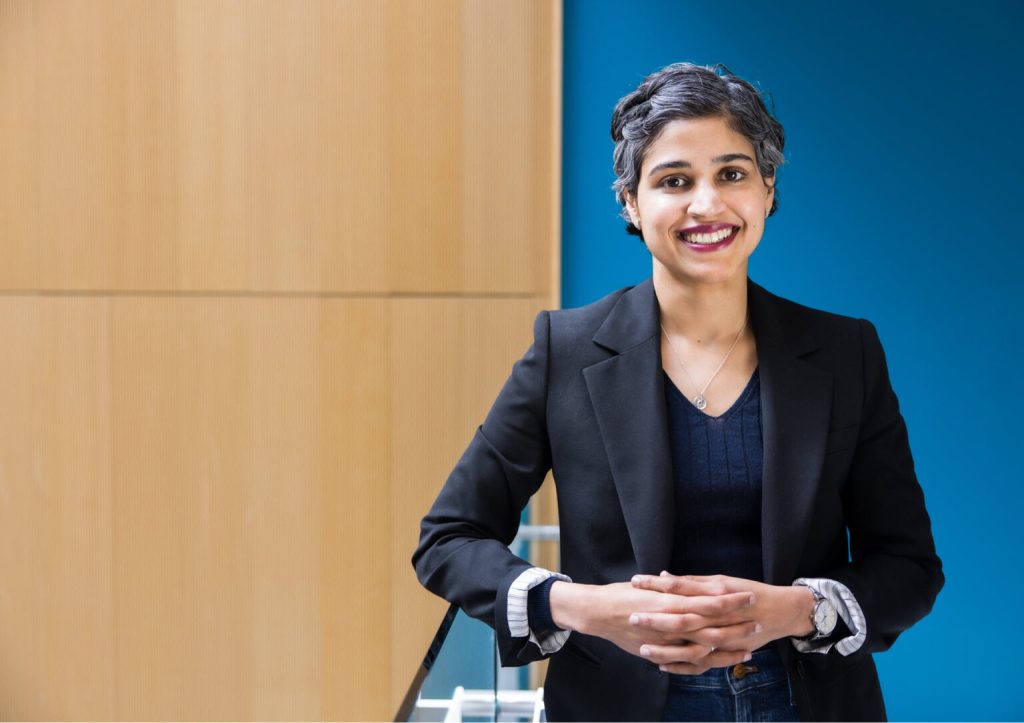
At the forefront of this breakthrough is the Phi-3-mini, a model measuring just 3.8 billion parameters – a mere fraction of the size of the largest language models. Yet, despite its diminutive stature, Phi-3-mini outperforms models twice its size across a variety of benchmarks, thanks to an innovative training approach developed by Microsoft researchers.
“Tiny but mighty: The Phi-3 small language models with big potential,” the blog post proclaims, encapsulating the essence of this technological marvel. “What we’re going to start to see is not a shift from large to small, but a shift from a singular category of models to a portfolio of models where customers get the ability to make a decision on what is the best model for their scenario,” said Sonali Yadav, principal product manager for Generative AI at Microsoft.
The key advantage of SLMs like Phi-3 lies in their ability to operate on devices with limited computational resources, enabling low-latency AI experiences without the need for constant network connectivity. “Some customers may only need small models, some will need big models and many are going to want to combine both in a variety of ways,” said Luis Vargas, vice president of AI at Microsoft.
Potential applications for these compact powerhouses are vast, ranging from smart sensors and cameras to farming equipment and remote monitoring systems. “If you are in a part of the world that doesn’t have a good network,” Vargas explained, “you are still going to be able to have AI experiences on your device.”
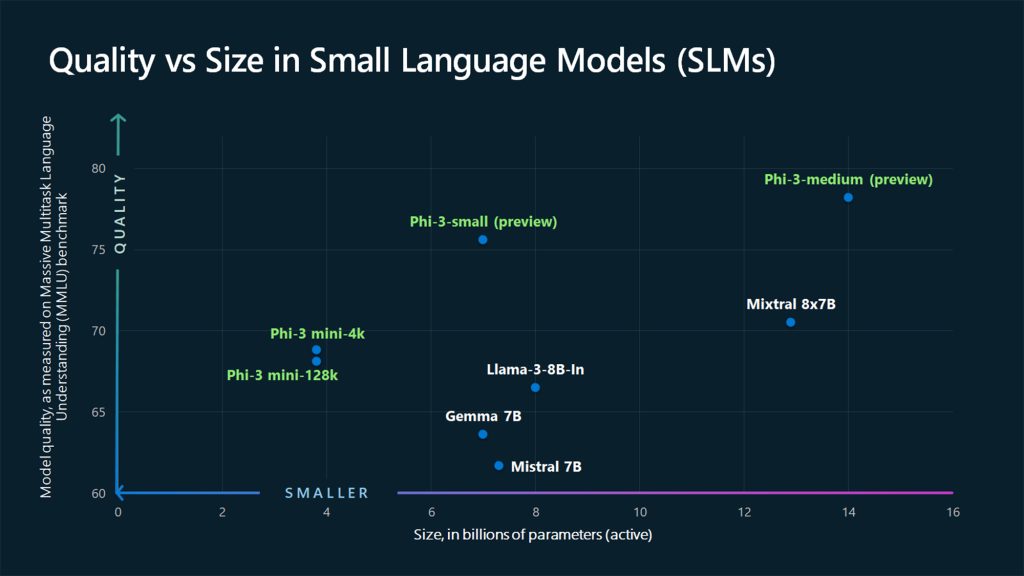
Moreover, by keeping data on the device, SLMs offer enhanced privacy – a critical consideration in an era where data breaches and cyber threats loom large. “Small language models also offer potential solutions for regulated industries and sectors that encounter situations where they need high quality results but want to keep data on their own premises,” Yadav noted.
While large language models (LLMs) remain the gold standard for complex reasoning tasks, such as drug discovery or comprehensive data analysis, SLMs provide a compelling alternative for simpler tasks like query answering, summarization, and content generation. “Anything that involves things like planning where you have a task, and the task is complicated enough that you need to figure out how to partition that task into a set of sub tasks, and sometimes sub-sub tasks, and then execute through all of those to come with a final answer … are really going to be in the domain of large models for a while,” Vargas acknowledged.
However, as Victor Botev, CTO and Co-Founder of Iris.ai, commented, “Rather than chasing ever-larger models, Microsoft is developing tools with more carefully curated data and specialized training. This allows for improved performance and reasoning abilities without the massive computational costs of models with trillions of parameters. Fulfilling this promise would mean tearing down a huge adoption barrier for businesses looking for AI solutions.”
The secret sauce behind the Phi-3 family’s exceptional performance lies in a groundbreaking training technique inspired by Eldan’s bedtime story realization. “Instead of training on just raw web data, why don’t you look for data which is of extremely high quality?” asked Sebastien Bubeck, Microsoft vice president of generative AI research who has led the company’s efforts to develop more capable small language models.
The researchers began by creating a discrete dataset dubbed “TinyStories,” comprising millions of simple narratives generated by prompting a large language model with combinations of words a 4-year-old would know. Remarkably, a model with just 10 million parameters, trained on this dataset, could generate fluent stories with perfect grammar.
Building upon this success, the team curated a larger dataset called “CodeTextbook,” comprising high-quality web data vetted for educational value. Through rounds of prompting, generation, and filtering by both humans and large AI models, the researchers synthesized a corpus of data tailored for training more capable SLMs.
“A lot of care goes into producing these synthetic data,” Bubeck emphasized. “We don’t take everything that we produce.” The high-quality training data proved transformative, as Bubeck explained: “Because it’s reading from textbook-like material, from quality documents that explain things very, very well, you make the task of the language model to read and understand this material much easier.”
While the thoughtful data curation process mitigates many potential risks, Microsoft emphasizes the importance of additional safety practices. “As with all generative AI model releases, Microsoft’s product and responsible AI teams used a multi-layered approach to manage and mitigate risks in developing Phi-3 models,” the blog post states.
This includes providing further training examples to reinforce expected behaviors, conducting assessments to identify vulnerabilities through red-teaming, and offering Azure AI tools for customers to build trustworthy applications atop Phi-3.
As the world embraces the transformative potential of artificial intelligence, Microsoft’s Phi-3 family represents a significant step toward democratizing this technology. By harnessing the power of simplicity and carefully curated data, the tech giant has demonstrated that size is not the sole determinant of capability in the realm of language models.
With the Phi-3-mini already available in the Microsoft Azure AI Model Catalog, Hugging Face, Ollama, and as an NVIDIA NIM microservice, and additional models like Phi-3-small and Phi-3-medium on the horizon, Microsoft is poised to empower a diverse range of organizations and individuals to harness the power of AI in ways that were previously unimaginable.
As Yadav aptly stated, “What we’re going to start to see is not a shift from large to small, but a shift from a singular category of models to a portfolio of models where customers get the ability to make a decision on what is the best model for their scenario.” With the Phi-3 family, Microsoft has taken a bold step toward realizing this vision, ushering in a new era of accessible, cost-effective, and privacy-conscious AI solutions for all.
Copyright©dhaka.ai
tags: Artificial Intelligence, Ai, Dhaka Ai, Ai In Bangladesh, Ai In Dhaka, OpenAI, ChatGPT, Meta, Google, Claude, Future of AI, Dhaka, Microsoft