Bioinformatics is an interdisciplinary field that uses computational and analytical methods to understand biological data. From analyzing DNA sequences to predicting protein structures, bioinformatics generates massive datasets that require robust computational tools to interpret. Artificial intelligence (AI) has emerged as a transformational technology in this regard with the ability to rapidly analyze complex biological data to uncover meaningful patterns.
The Current Landscape of AI in Bioinformatics
AI adoption in bioinformatics is accelerating across diverse domains like genomics, imaging, drug discovery and healthcare analytics. In genomics, AI techniques like machine learning and deep learning enable high-throughput annotation and analysis of genomic data [1]. For instance, tools like DeepVariant and DRAGEN incorporate neural networks for variant detection in next-generation sequencing data [2]. Such innovations are vital for breakthroughs in areas like personalized medicine.
Likewise, sophisticated reinforcement learning algorithms empower systems like Rosetta to predict protein structures which serves as the basis for rational drug design [3]. Companies like Insitro and BenevolentAI are actively utilizing AI to quicken the drug discovery pipeline by uncovering novel biological targets [2]. Digital pathology powered by AI also helps enhance disease diagnosis, as demonstrated by startups like PathAI using deep learning for cancer detection [2]. Medical imaging sees vast applications of AI with companies like Zebra Medical Vision harnessing computer vision for advanced radiology techniques.
Evidently, AI integration in bioinformatics encompasses a diverse spectrum spanning genomic medicine, molecular modeling, pharmaceuticals and clinical practice. What unites these varied use cases is the ability of AI to rapidly analyze complex biomedical data to generate actionable knowledge. And this is just the beginning of AI’s foray into bioinformatics. Exciting innovations lie ahead.
The Road Ahead – Three Emerging Trends
Integrated Multi-Omics Frameworks
As high-throughput omics technologies like genomics, transcriptomics and metabolomics continue maturing, systems-based approaches that can integrate and jointly analyze different omics data types are indispensable to understand interconnections underlying biological phenomena. AI models that fuse cross-omics data to model molecular interactions within the context of cellular states and physiological characteristics will prove invaluable in this regard [4]. Rather than analyzing omics datasets in silos, joint multi-omics AI models need to become ubiquitous.
Enabling Personalized and Preventive Healthcare
An emerging emphasis on predictive, preventive, personalized and participatory (P4) medicine necessitates assimilating diverse patient data encompassing genomics, electronic health records, wearable sensors etc. to enable risk stratification, early diagnosis and tailored interventions [5]. AI will be pivotal in securely integrating such multi-parametric data into actionable individualized care pathways while preserving patient privacy. Startups like Tempus and SOPHiA Genetics already offer such AI-powered solutions but more scalable medical-grade standards are warranted for clinical reliability assurance [2].
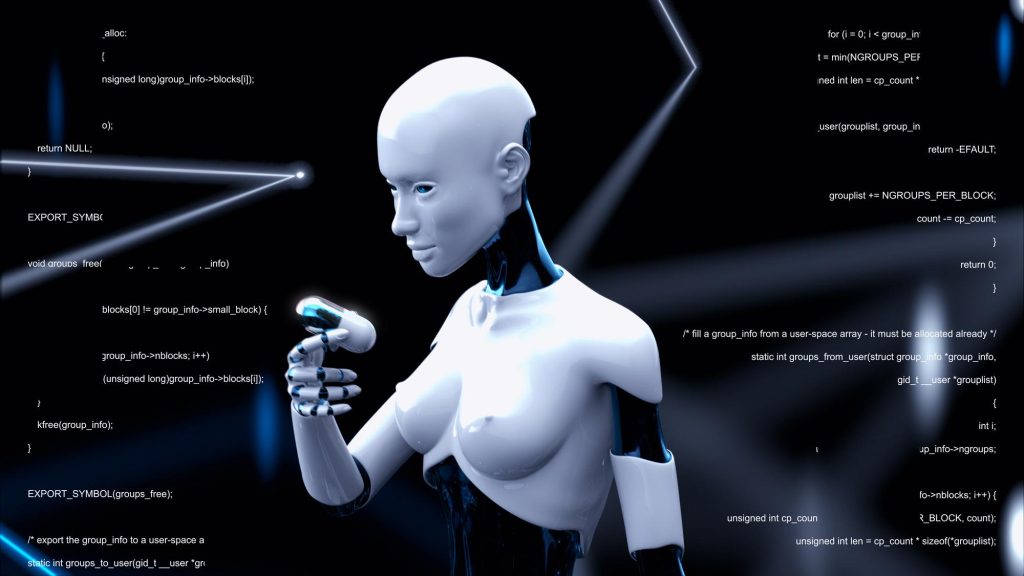
Revolutionizing Scientific Discovery
While currently AI in bioinformatics supports analyzing existing datasets, the next frontier entails AI initiating hypotheses and scientific discovery autonomously by deducing non-intuitive patterns across multi-omics data. Instead of just confirming known biology statistically, AI models need to progress towards revealing novel biology not conceivable via status quo human-led research. The application of generative deep learning methods appears particularly promising to realize biomarker discoveries and unearth fresh perspectives. Such AI-driven biological research can help unleash data-led serendipities!
Strategies for Incorporating AI into Bioinformatics
Given the tremendous promise of AI in transforming bioinformatics, what should computational biologists do to avail such innovations? Here are five practical suggestions:
- Explore Online Tutorials: Numerous universities and MOOC platforms like Coursera offer beginner-friendly courses on AI/ML for biologists without intensive programming needs. These are great starting points.
- Seek Expert Mentorship: Reach out to developers of tools like CellProfiler to learn implementational best practices from peer pioneers. The open-science community is welcoming of queries.
- Upskill in Data Science: Complement domain expertise with data analytics skills – basics of Python, statistics and software engineering – to customize tools for research contexts.
- Leverage Pre-Trained Models: With libraries like TensorFlow and PyTorch, pre-made ML models for bio-applications can be readily adapted instead of coding solutions from scratch.
- Join Online Communities: For troubleshooting or sharing ideas, participate in forums like BioStars and Image.sc that host discussions on AI adoption in life sciences.
By taking such proactive measures, computational biologists can smoothly onboard state-of-the-art AI innovations into their workflows and reap associated benefits ranging from accelerated discoveries to improved data analysis rigors. Collaborating with data scientists also helps overcome technical hurdles for seamless translation of AI utilities into practical biological insights.
Promoting Responsible and Ethical AI Innovation
However, in the rush to capitalize on AI’s potential, upholding responsible and ethical standards is equally important, especially for sensitive translation of technologies into clinical practice [6]. Some crucial considerations in this context include:
Ensuring Representativeness of Training Data: Algorithmic biases can sneak in if AI models are trained on incomplete or skewed datasets not accurately representing diverse human populations and health conditions. Hence curating comprehensive and balanced training data should remain a priority.
Evaluating Algorithmic Accountability: Stringent bench-marking on safety parameters is vital before deploying AI diagnostics or prediction tools for actual patient care. Failure modes of AI should be well-characterized.
Promoting Fairness, Transparency and Interpretability: As AI permeates high-stakes domains like healthcare, ensuring equitable access along with transparency regarding data provenance and algorithmic interpretability becomes imperative.
Advocating Data Protection and Privacy: For healthcare settings, adequate consent, anonymization, encryption and cybersecurity mechanisms for patient data used to develop AI need to be instituted to maintain public trust and credibility.
By self-regulating around such ethical AI design principles, the bioinformatics community can nurture cutting-edge innovations responsibly.
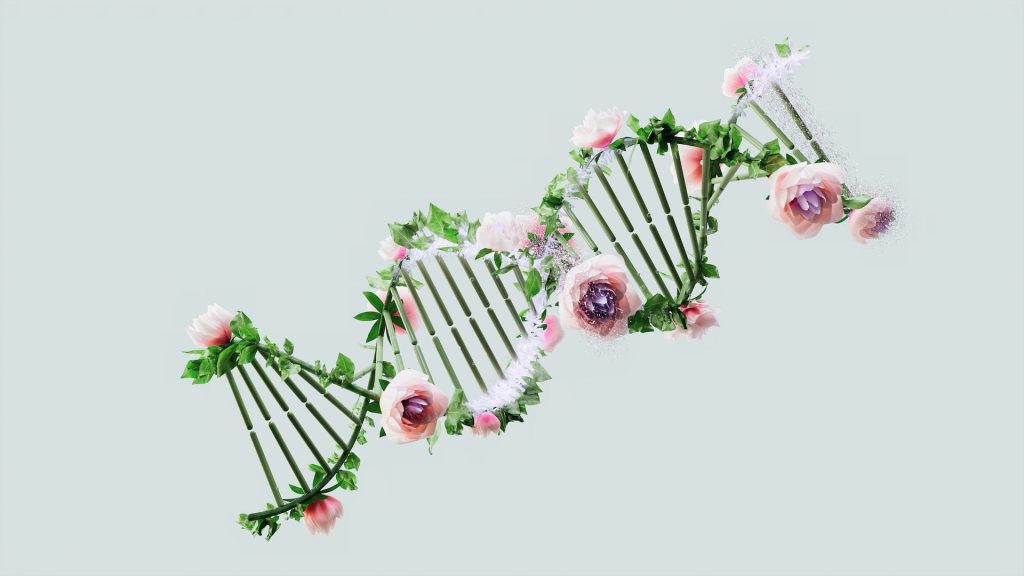
Wider Implications for Science and Medicine
The application of AI in bioinformatics heralds tremendous promise to transform biological research and medical practice by enhancing the ability to manage complex datasets, automate analytical workflows and generate actionable diagnostics. Besides efficiency gains, AI can reveal novel insights challenging status quo knowledge – thereby initiating scientific paradigm shifts rather than just confirming existing hypotheses.
Furthermore, AI-driven analytical tools promise to democratize state-of-the-art biomedicine by increasing access to advanced diagnostics and enabling individualized care independent of geographic constraints. Such technologies also allow closer patient participation and self-driven health management.
Thus with astounding growth anticipated in biotechnology, bioinformatics integrated with AI looks destined to form the foundational fabric supporting modern data-driven, preventive and precision medicine tailored to each person’s unique disease risks, prognosis and treatment response. This confluence of scaled biology, scaled computing and scaled access facilitated by AI constitutes the next big leap for human health and longevity!
The future of bioinformatics being shaped by AI indeed looks exciting. By adopting a judicious and ethical approach, the biomedical community is well poised to harness innovations in this space responsibly for accelerating discoveries that matter to patients.
References
[1] Ezziane, Z. (2006). Applications of artificial intelligence in bioinformatics: A review. Expert Systems with Applications, 30(1), 2–10.
[2] Kumar Panda, P. (2023). Discover 50 AI-Powered Tools Shaping the Future of Bioinformatics!
[3] Cohen, J. (2004). Bioinformatics-an introduction for computer scientists. ACM Computing Surveys, 36(2), 122-158.
[4] Gligorijević, V., Malod-Dognin, N. & Pržulj, N. Integrative methods for analyzing big data in precision medicine. Proteomics 16, 741–758 (2016).
[5] Char, D. S., Shah, N. H., & Magnus, D. (2018). Implementing machine learning in health care—addressing ethical challenges. The New England journal of medicine, 378(11), 981.
[6] Bayat, A. (2002). Bioinformatics. BMJ (Clinical research ed.), 324(7344), 1018–1022.
Copyright©dhaka.ai
tags: Artificial Intelligence, Ai, Dhaka Ai, Ai In Bangladesh, Ai In Dhaka, USA